Researcher Interviews
A research vision to enhance video understanding and knowledge conversion - breakthrough in long-term video analysis to support the video analytics AI agent
JapaneseThe video analytics AI agent, announced by Fujitsu in December 2024, is a technology that autonomously supports on-site work by utilizing work-related videos and documents. The aim is to improve work efficiency and create safer and more secure work environments. To support this agent’s future video understanding and memory capabilities, we are engaged in the R&D of long-term video analysis. This technology converts long-term videos into compact memory and graph data, enabling efficient search and analysis through question-and-answer interaction via a chatbot. Long-term video analysis contributes to solving various business challenges, such as identifying accident risks, analyzing worker behavior, and verifying the effectiveness of product layout changes. It does this by enabling interactive analysis of long-term video data such as surveillance camera footage from warehouses and factories, which was previously difficult to utilize due to the challenges of tagging and classification. In this article, we interviewed six members of the long-term video analysis research team to learn more about the background of the technology's development and the challenges they faced.
Published on February 28, 2025
MEMBERS
-
Sosuke Yamao
Principal Researcher
Human Reasoning Core Project
Artificial Intelligence Laboratory
Fujitsu Research
Fujitsu Limited -
Takashi Honda
Research Director
Human Reasoning Core Project
Artificial Intelligence Laboratory
Fujitsu Research
Fujitsu Limited -
Junya Saito
Principal Researcher
Human Reasoning Core Project
Artificial Intelligence Laboratory
Fujitsu Research
Fujitsu Limited -
Shingo Hironaka
System Engineer
Optical Solution Business Division
Photonics System Business Unit
System Platform Business
Fujitsu Limited -
Arisu Endo
Researcher
Human Reasoning Core Project
Artificial Intelligence Laboratory
Fujitsu Research
Fujitsu Limited -
Natsuki Miyahara
Researcher
Human Reasoning Core Project
Artificial Intelligence Laboratory
Fujitsu Research
Fujitsu Limited
Deriving insights from long-term video analysis
What is the purpose of developing long-term video analysis?
Takashi: The goal of long-term video analysis is to support business operations by providing relevant information and actionable strategies tailored to the customer's environment, based on long-term, large volume video data. Let me illustrate this with a specific example involving the analysis of customer purchasing behavior. Suppose sales of product X are stagnant. By analyzing in-store camera footage through long-term video analysis, we can identify customer behavior patterns and support the development of measures to improve sales. For instance, if we ask, "Please show the actions of customers, who picked up product X but did not purchase it, along with the context before and after, in chronological order," the system analyzes past footage and provides answers such as, "There were 20 instances in the past week where customers picked up product X but did not purchase it. In 15 of those instances, they checked the price display and then returned the product to the shelf." In this way, long-term video analysis allows us to analyze customer behavior in detail, uncovering insights that were often overlooked before, and enabling us to take appropriate action.
What technologies comprise long-term video analysis?
Takashi: It consists of two key technologies: First, video context memory technology, which enables efficient video storage by selecting important information from long-term videos and storing only that information. Second, Fujitsu Knowledge Graph Enhanced Retrieval Augmented Generation (RAG) for Vision Analytics, a technology that structures complex video information chronologically into a graph structure, allowing appropriate answers to be presented to the user instantly. Additionally, we are collaborating with the Fujitsu Macquarie AI Research Lab (one of the Fujitsu Small Research Labs), established at Macquarie University, which is engaged in various AI research projects aimed at solving social issues. This collaboration facilitates field trials and technological development.
What prompted the development of long-term video analysis?
Takashi: Fujitsu has cultivated various video recognition technologies, such as "Actlyzer," an AI that recognizes a variety of human actions from video, and "Fujitsu Markerless Motion Capture," used in gymnastics scoring systems. Long-term video analysis was developed by leveraging the experience gained from developing these technologies. By combining it with generative AI, which has shown remarkable growth in recent years, we aim to enhance the video analysis capabilities of the future video analytics AI agent, thereby supporting customers in improving their operational efficiency and productivity.
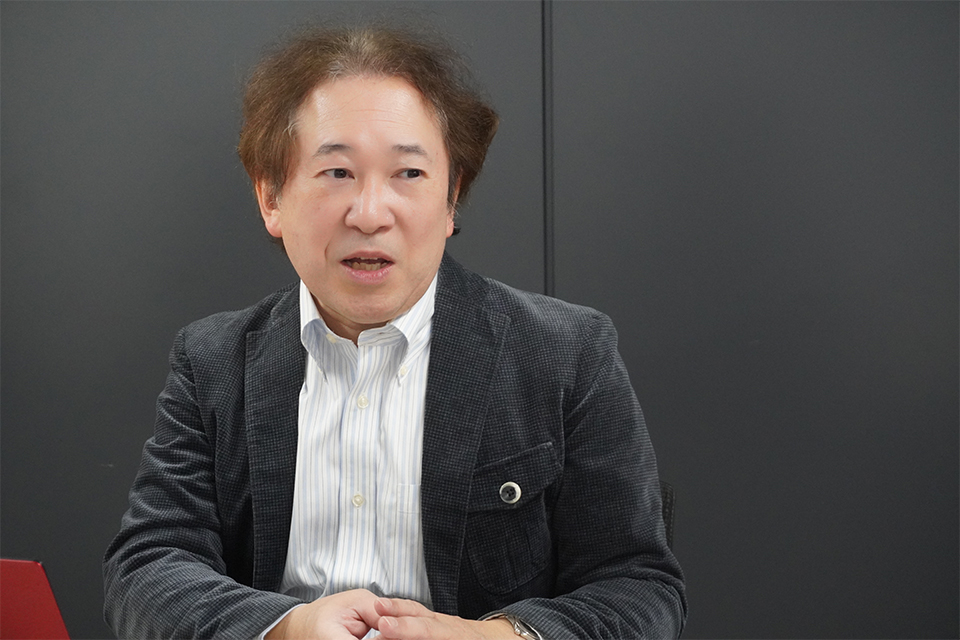
From detecting dangerous actions in factories to occupational health and safety management
How would the use cases and applications expand if the AI agent could analyze long-term video?
Sosuke: If the AI agent could analyze long-term video, use cases would expand to various fields, such as occupational health and safety management in factories and commercial facilities, as well as infrastructure maintenance and inspection. Currently, many sites accumulate video data captured over long periods at multiple locations, but utilizing this vast amount of data has been difficult. If long-term video analysis by the AI agent become possible, it will enable autonomous operational support based on this video data, contributing to solving previously unaddressed challenges. For the AI agent to acquire such video analysis capabilities, we need to overcome challenging technical hurdles, such as accurate understanding and memorization of long-term video, together with the conversion and utilization of video content into knowledge. We are working on developing long-term video analysis to address these technical challenges in collaboration with professors and students at Macquarie University, and we are also conducting trial experiments at a FAL (Fujitsu Australia Ltd.) warehouse.
Could you elaborate on the trial experiment?
Arisu: For example, let's say we want automatically to detect dangerous scenes from video footage, such as a forklift moving towards a worker in a warehouse. We input the target video for long-term video analysis and ask via chatbot, "Were there any instances where a forklift approached a worker? Was the worker wearing a safety vest at that time?" The system then extracts the times when the forklift and worker were close, and the worker's safety vest wearing status from the video, then providing the answers. While it's tedious for humans to review long videos, long-term video analysis allows us to obtain detailed chronological information through dialogue. By asking questions like, "What happened before this action?" or "Tell me what the person did afterward," we can easily understand the context surrounding an event. This allows on-site personnel to gain a deeper understanding of the situation and more easily devise countermeasures. In the field trials, we were able to detect dangerous actions from actual warehouse operation videos, and by incorporating knowledge related to occupational health and safety management, we were able to propose potential improvements.
Creating value that leads to solving social issues through industry-academia collaboration
How significant is the joint research with Macquarie University?
Arisu: Not just warehouses, but every site wanting to utilize AI has different environments and rules. Therefore, instead of a conversational AI that provides generic answers, you need personalization tailored to the specific site. By combining Macquarie University's expertise in personalization with Fujitsu's technology, we can adapt long-term video analysis to each individual site. Also, Macquarie University has expertise in coaching, so we believe this will allow us to serve our customers better, for example, by guiding on-site workers towards safer actions.
We heard that some researchers were dispatched to the Fujitsu Macquarie AI Research Lab to strengthen the relationship.
Sosuke: There are things you can only understand through face-to-face interaction. By having researchers stationed there and understanding each other's research strengths, we can collaborate more effectively. While online connections are important, there's a lot to be gained from actually visiting and engaging in dialogue, which strengthens relationships. We believe it's important to have multiple points of contact. We want to go beyond our current joint research and explore the potential of our respective technologies further, developing new joint research projects to open up new application areas and overcome technical challenges.
What are some potential future use cases and on-site applications for this technology?
Takashi: First, we want to be able to visualize the coaching effects on workers and propose improvements in settings like warehouses. We are also considering applications in the medical field, particularly in rehabilitation. Macquarie University has a university hospital equipped with state-of-the-art facilities and extensive expertise in coaching within medical settings. Through collaboration with the university, we plan to develop this technology further into a more practical tool while utilizing it in real-world environments.
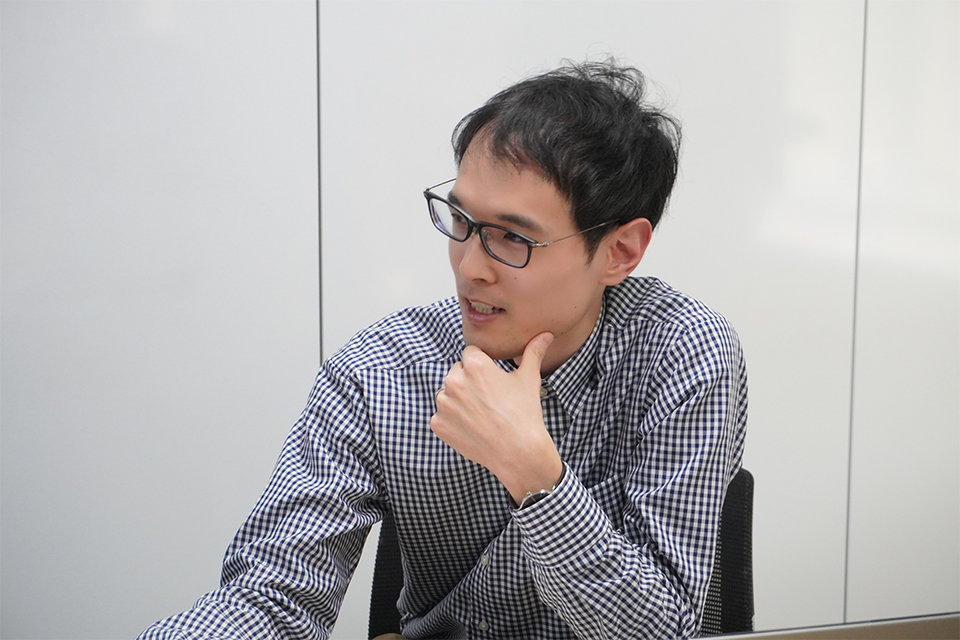
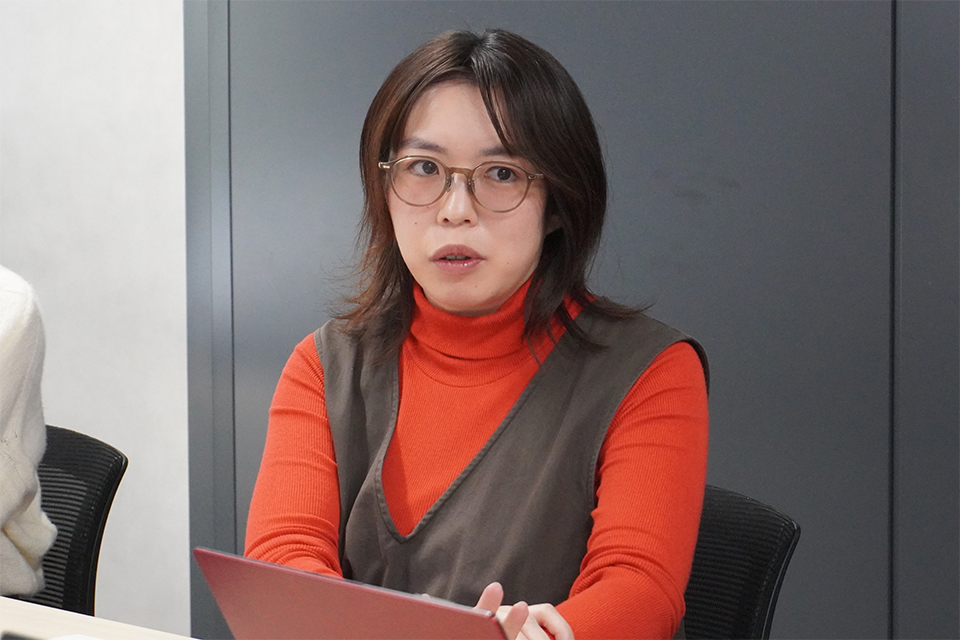
Fujitsu Knowledge Graph Enhanced RAG for Vison Analytics: Enabling question answering based on video-generated knowledge graphs
The research team is developing two core technologies for long-term video analysis: Knowledge Graph Enhanced RAG for Vision Analytics and video context memory technology. We interviewed the research team responsible for these developments, asking them about the strengths of each technology and the challenges faced during development. The first technology we will introduce is Knowledge Graph Enhanced RAG for Vision Analytics, a technology capable of identifying events such as dangerous actions from video data and analyzing their frequency and trends.
Tell us about the strengths and characteristics of Knowledge Graph Enhanced RAG for Vision Analytics.
Junya: Knowledge Graph Enhanced RAG for Vision Analytics’ strength lies in its ability to answer questions using a knowledge graph generated from video. Accurate answers can be obtained even from massive amounts of video data. While current generative AI can recognize general information about what's shown in a video when asked questions, it cannot accurately answer questions about the chronological order of events or detailed information. Our technology overcomes these challenges and enables highly accurate answers. Importantly, our approach of generating and analyzing a knowledge graph that shows the relationships between people and events in a video sets us apart from other companies' large language model (LLM) development.
Who are your target users for this technology?
Junya: We envision this technology being used by managers in charge of product placement, equipment inspection, and manufacturing operations in settings such as retail stores and manufacturing sites. We hope that it will be helpful for analyzing the current situation based on on-site video footage and formulating measures to improve operational efficiency and reduce human error.
What were the challenges in developing this technology, and how did you overcome them?
Shingo: As the amount of data in the knowledge graph increases and it grows larger, the AI's processing time increases, leading to slower responses and sometimes incorrect answers. This is because AI changes its calculation methods each time, so processing time and the consistency of results are not guaranteed. To address this challenge, we repeated the process of prototype development and improvement. While conventional AI processing is a black box with unclear mechanisms, we devised a method that combines highly reliable algorithms designed for specific problem-solving. This enables fast, accurate, and consistent answers.
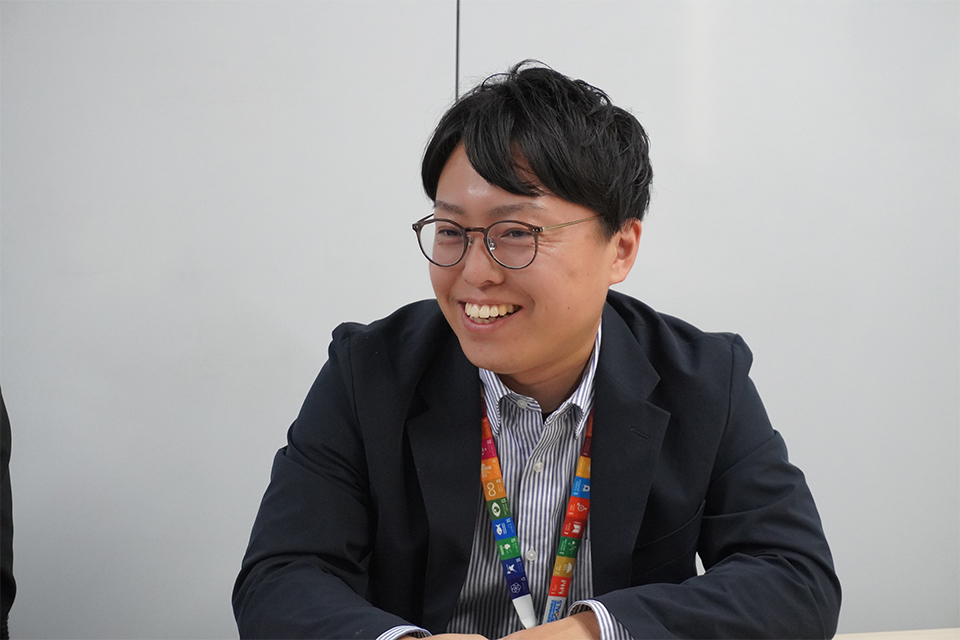
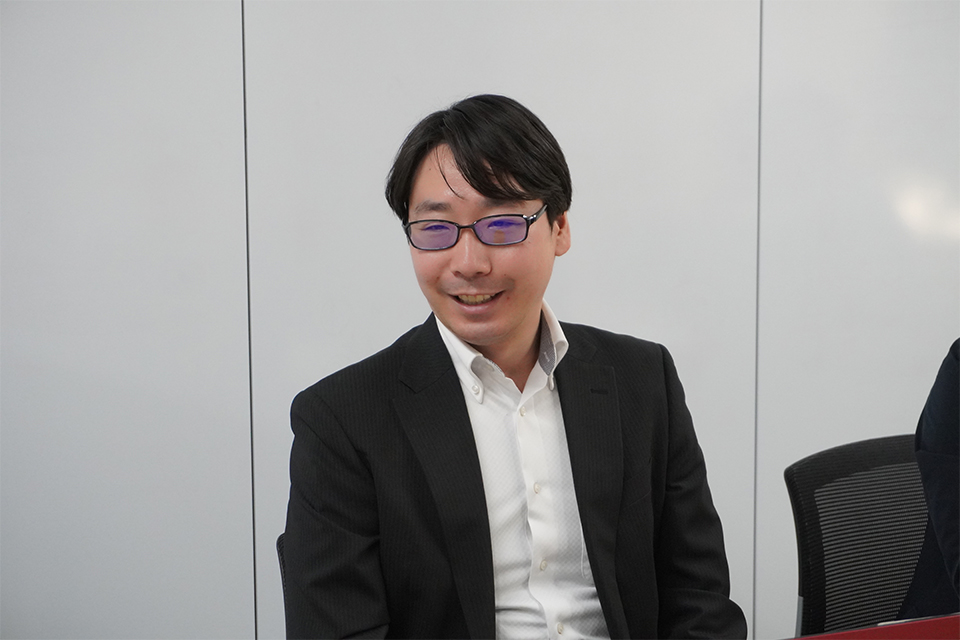
A new video context memory technology inspired by selective attention and memory
On-site work video data can amount to enormous volumes, sometimes spanning several hours. This is where video context memory technology, one of the core technologies of long-term video analysis, plays a crucial role. This technology selectively stores important information from long-term videos. While Fujitsu Knowledge Graph Enhanced RAG for Vision Analytics can structure complex video information chronologically into a graph format and instantly provide appropriate answers to user questions, this video context memory technology is essential for efficiently processing vast amounts of video data.
Tell us about the strengths and characteristics of video context memory technology.
Sosuke: Video context memory technology is based on a new video understanding paradigm inspired by the human cognitive characteristics of selective attention and memory. Just like in the "Invisible Gorilla (*1)" experiment, when humans concentrate on a specific task while watching a video, they intentionally overlook information irrelevant to the task, while efficiently memorizing important information related to it. Based on this characteristic, we devised a technology that selectively extracts only the video information crucial for question answering and analysis, and efficiently stores it in memory using minimal capacity. While conventional technologies store entire long-term videos, our technology pre-assigns a task to the AI, such as identifying characters, situations, or objectives, allowing it to extract and store only necessary information, achieving both high memory efficiency and answer accuracy. In a video understanding benchmark (*2) including long-term videos exceeding one hour, which are difficult for existing technologies to process, we achieved higher memory efficiency and answer accuracy than state-of-the-art methods.
Who are your target users for this technology?
Sosuke: We envision this technology being used by those who want to analyze and utilize long-term video data that is difficult to handle with existing technologies, such as multi-location, long-term surveillance camera footage from factories, commercial facilities, and urban areas, as well as video content like movies and dramas. Many businesses possess long-term videos but struggle to utilize them effectively. Of course, individuals may also face similar challenges. As researchers, we hope this technology will be widely used, and not limited to just specific fields.
Natsuki: Use cases for analyzing accidents on-site are also conceivable. It's important to grasp not only the detection of the accident itself but also the events leading up to it. It's difficult to understand the process with only discrete data. We expect this technology will enable tracking the actions of individuals involved in accidents and help to clarify the causes and circumstances.
What were the challenges in developing this technology, and how did you overcome them?
Natsuki: If we try to make AI memorize entire long-term videos, the storage capacity of the recording device becomes a limiting factor, leading to data overflow and the inability to store everything. Therefore, it's necessary to store videos efficiently to fit within the computer's specifications. We thoroughly gathered information on existing data compression and recording methods and repeatedly discussed various ideas. As a result, we arrived at a method of efficient storage using a compression technique inspired by human memory, which focuses on the necessary context.
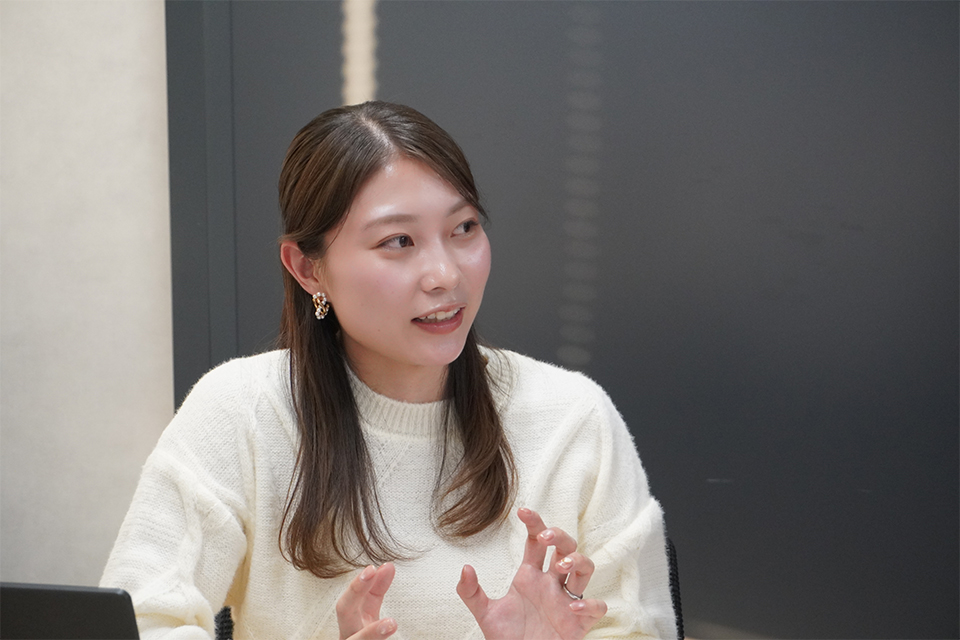
Behind the scenes of long-term video analysis development
How was the long-term video analysis development team formed?
Takashi: In the research unit, the basic approach is for each member to have their own research theme and delve into it. However, recently there has been a movement to bring together individual technologies and skills to aim for greater achievements. The long-term video analysis project is one example of this, and it can be considered a new initiative in that researchers have come together to tackle a new theme and have produced significant results. Many team members joined through Fujitsu's career support system, including internal postings and the "Job Challenge!!" (a temporary transfer program within Fujitsu lasting 3-6 months, designed to broaden horizons and explore career options).
Could you tell us about the research process?
Natsuki: This team consists of members with diverse skills and backgrounds. Therefore, we believe face-to-face communication is essential. While we usually work remotely, we have regular co-working days where everyone gathers together. By sharing information that is difficult to convey remotely and actively discussing ideas using a whiteboard, we maintain close communication.
What enabled the team to release the technology in such a short timeframe?
Shingo: In recent years, the pace of technological innovation in AI, particularly LLMs, has been extremely rapid, and swift development is required to stay ahead of competitors. Therefore, we assembled members with individual strengths, such as chat system development, user interface design, and knowledge graph expertise, and combined our knowledge to advance development. To proceed with the cycle from planning to development within a short period, the entire team worked together under an agreed-upon schedule. By sharing development progress through videos and code, and rapidly iterating feedback, we were able to finalize the final vision early on and minimize revisions in later stages.
Where can people experience this technology?
Shingo: If you are interested in the long-term video analysis and its core technology, Knowledge Graph Enhanced RAG for Vision Analytics, please contact us. We are also preparing to provide an environment where you can experience video context memory technology. For information on other advanced AI technologies from Fujitsu, please visit the Fujitsu Kozuchi website.
Researchers envision the future, preparing for the age of AI
What are your future plans for the video analytics AI agent and long-term video analysis?
Sosuke: Research on long-term video analysis is a fascinating theme that delves into the essence of human vision and intelligence. It explores the core of the video analytics AI agent, including semantic understanding and reasoning based on visual information, short-term and long-term video memory, together with planning and actions based on those memories. Moreover, video context memory technology has the potential to be a real breakthrough, enabling AI agents to acquire the quintessential human cognitive trait of selective attention and memory. We expect our efforts to contribute significantly to the technological development and industrial applications of AI agents and multi-AI agent systems, which are likely to become future trends.
Junya: User-friendliness is crucial. With the video analytics AI agent, we aim for a system that minimizes user effort, enabling video recognition and analysis solely through text input. Of course, we don't expect to achieve 100% accuracy at this stage. However, we believe we can make it better through ingenuity. We are aiming for a system that is easy for anyone to use, with simple operations that can improve accuracy even without specialized knowledge, and customization options for experts to enable advanced recognition and analysis.
Shingo: Fujitsu possesses technology for the detailed recognition of human movement, accumulated through the development of the Judging Support System for gymnastics. By utilizing this technology, we want to expand the range of applications by enabling the system to answer questions about workloads in factories and physical strain in sports.
Arisu: We are considering expanding the application of this technology by promoting its integration with business systems and collaborating with other teams working on AI technologies in different fields. The video analytics AI agent can be combined with various technologies and industries. We want to continue enhancing this technology and encourage its wide use through co-creation and collaboration.
Natsuki: With its advancements, AI has become capable of handling diverse data such as text, images, audio, and video, and understanding their content. In the future, it will be required to process even larger amounts of data. By further developing the processing and recording efficiency we are working on, we believe the scope of application for the video analytics AI agent will continue to expand.
Takashi: This technology is attracting interest both inside and outside the company. Its appeal lies in its ability to recognize not only people in videos but also various objects and easily extract necessary information through dialogue using LLMs. Going forward, we aim to expand its applicability and evolve it into a system that allows for easy customization and updates without specialized knowledge such as programming.
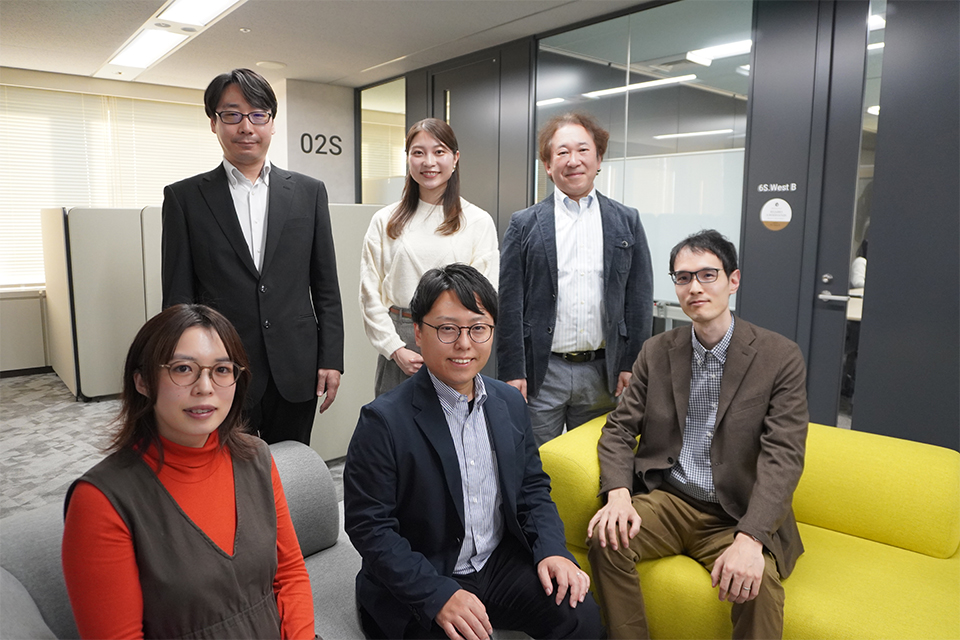
-
(*1)The "Invisible Gorilla” experiment demonstrates the selective nature of human attention. Participants are instructed to count the number of passes made by players wearing white shirts in a video. However, many participants fail to notice a person in a gorilla suit walking across the center of the screen.
-
(*2)This benchmark utilizes a subset of 599 questions (with videos averaging 49 minutes and a maximum of 151 minutes) from InfiniBench, a state-of-the-art benchmark designed to evaluate long-term video understanding performance, specifically those answerable solely from the video information.
Fujitsu's Commitment to the Sustainable Development Goals (SDGs)
The Sustainable Development Goals (SDGs) adopted by the United Nations in 2015 represent a set of common goals to be achieved worldwide by 2030. Fujitsu's purpose — “to make the world more sustainable by building trust in society through innovation”—is a promise to contribute to the vision of a better future empowered by the SDGs.
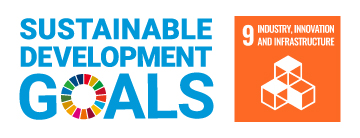