Researcher Interviews
Talking to the team behind Fujitsu Kozuchi (code name) - Fujitsu AI PlatformWorking to accelerate the social implementation of AI (Operation Process Analysis & Product Defect Inspection chapter)
JapaneseIn recent years, AI is playing an increasingly important role in improving and expanding business operations. According to a survey conducted by Fujitsu, 67% of organizations said they will increase their investment in AI over the next five years (*1). There have been many breakthroughs in the field of AI one after another, and various industries, including manufacturing, retail, finance, and healthcare, are all focusing on AI applications as a priority. However, despite all of this activity, there are still many cases where AI has not been fully adopted, and challenges remain with regard to implementing AI in actual systems. Fujitsu is tackling this problem head on with the release of the "Fujitsu Kozuchi (code name) - Fujitsu AI Platform" (*2), an AI platform that enables the rapid application and verification of advanced AI developed by Fujitsu. In this interview, we talk to two key members of the research team responsible for the AI Innovation Components of the platform, namely Operation Process Analysis and Product Defect Inspection for the manufacturing industry. A component is a package of AI technologies needed to solve a problem. In this interview, we talk to them about their R&D efforts to solve problems in the manufacturing industry and how their research contributes to the realization of a sustainable society.
Published on September 22, 2023
MEMBERS
-
Atsunori Moteki
Researcher
Human Reasoning Core Project
Artificial Intelligence Laboratory
Fujitsu Research
Fujitsu Limited -
Shunichi Watanabe
Researcher
AI Innovation Core Project
Artificial Intelligence Laboratory
Fujitsu Research
Fujitsu Limited
Initiatives to realize digital transformation in manufacturing
What challenges do Operation Process Analysis and Product Defect Inspection solve on the manufacturing floor?
Atsunori (Operation Process Analysis): Our customers in the manufacturing industry need to analyze the work of each worker in order to improve the efficiency of their manufacturing sites and to pass on skills. However, in the past, it was necessary for a supervisor to observe and analyze the work as it was done, and then measure the time required with a stopwatch or other device, involving a time-consuming and costly exercise. The Operation Process Analysis component can automatically estimate the type of work being performed by workers based on camera images. As a result, it can optimize the manufacturing site by recognizing and eliminating wasteful work, noting any variations between workers, and identifying potentially dangerous postures that could lead to accidents and injuries, while reducing costs at the same time.
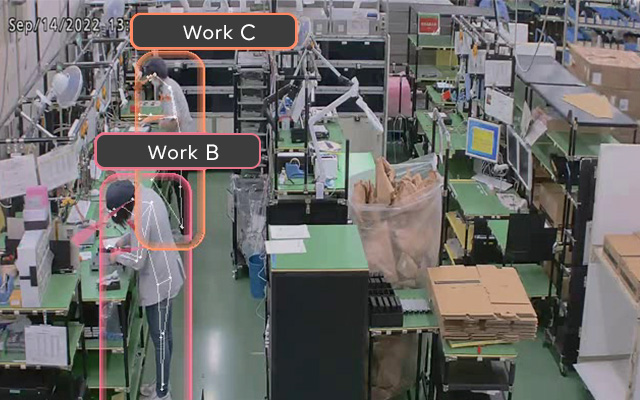
Shunichi (Product Defect Inspection): In the manufacturing industry, failing to identify a defective product can severely impact on user confidence. With our approach, it is possible to detect defective products from image data with high accuracy, thereby reducing the risk of defective products leaking in to the marketplace. When creating an AI model to detect defective products, it is common practice to use both good and defective product data. However, in actual manufacturing sites, defective product data is difficult to obtain, and it takes time to secure sufficient data. Therefore, we have developed a technology to construct a highly accurate AI model using only good data, constantly estimating and monitoring the accuracy of the AI model and automatically repairing the model when the accuracy tends to decline. This is important as the accuracy of the AI model may decline due to changes in the on-site environment. These technologies are aimed at realizing the improved operational stability of AI in Product Defect Inspection.
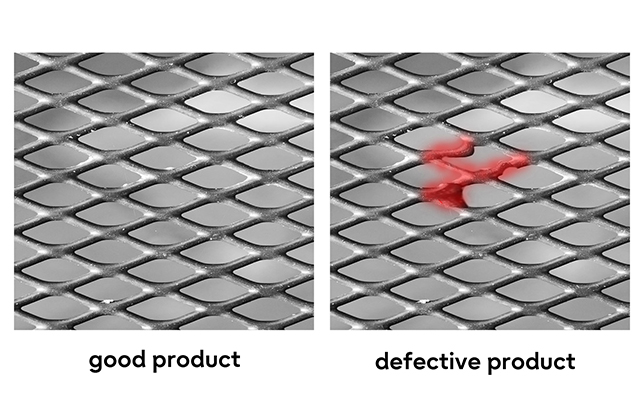
What technologies are used in the Operation Process Analysis and Product Defect Inspection components?
Atsunori: The Operation Process Analysis component uses Actlyzer (*3), a behavior analysis technology that recognizes human behavior from camera images. It is capable of recognizing approximately 100 basic behaviors that have been learned in advance, and, can also recognize complex behaviors by combining basic behaviors. For example, a "Smartphone Zombie" can be defined and recognized as a combination of the basic actions of "walking," "face down," and "arms forward”. In this way, detailed rules for work can be set according to the actual site situation. Real-time images from cameras installed at the work site can be used to analyze worker behavior, such as measuring work time and confirming work procedures. In addition, by recording the timing and location of falls and potentially dangerous postures, etc., and identifying on which tasks they occur, it is possible to identify risks based on an understanding of the current situation.
Shunichi: The Product Defect Inspection component uses two main technologies. First is the Defect Removing AutoEncoder (DeRA) (*4), an image AI for Product Defect Inspection, which creates AI models using only images of good products to detect various product defects and accurately locate them. The second is High Durability Learning (HDL) High Durability Technology (*5), which is responsible for the stable operation of AI. This technology can automatically control the degradation of AI accuracy when environmental or operational data changes that cause AI model degradation occur, thereby helping to solve the problem of AI accuracy degradation during operation. These technologies can continue to detect defective products generated in the manufacturing process with high accuracy, reducing the risk of outflow to the market due to missed products, as well as improving product productivity and quality.
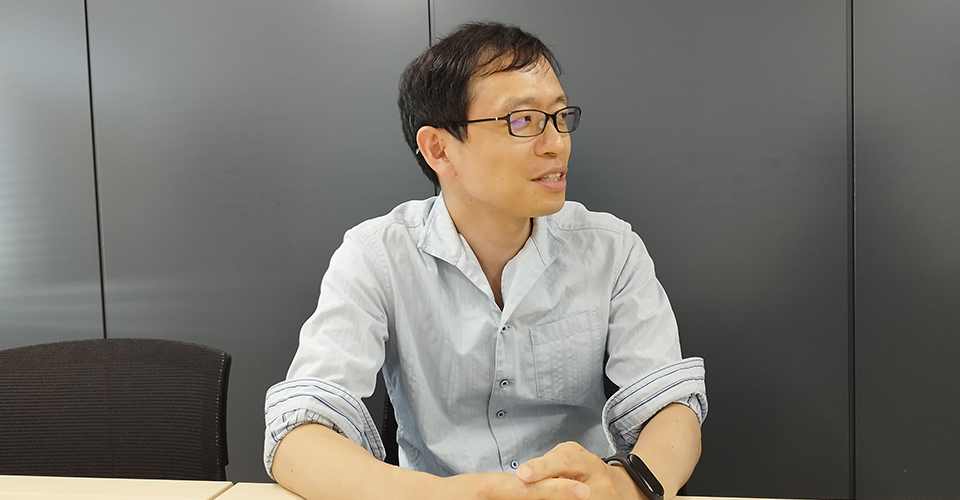
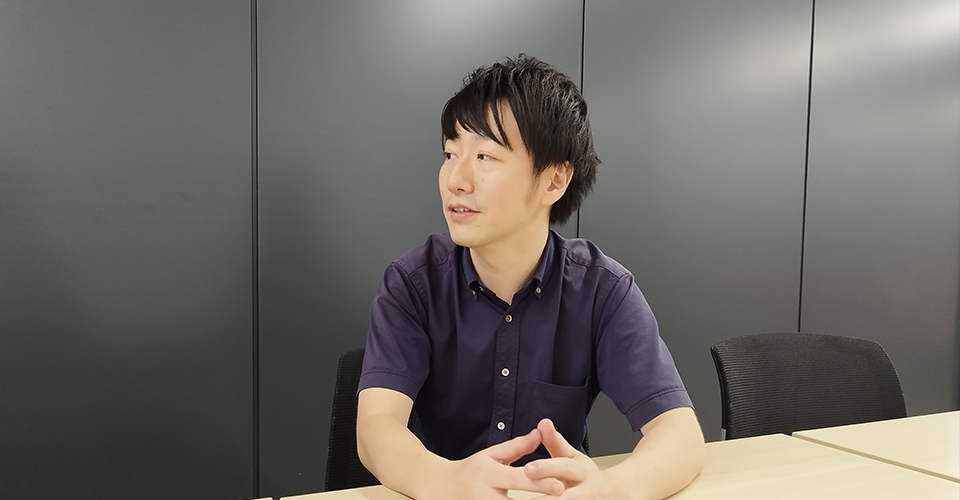
What are the features of Actlyzer, DeRA and HDL technologies respectively?
Atsunori: Actlyzer won first place in Papers With Code, the well-known benchmark in the machine learning field, and was also accepted at the top conferences ICPR2020 (*6), ICPR2022 and ICIP2022 (*7). It also won second place in the open competition for multi-person tracking technology at ECCV2022 (*8). The reason for the explosive interest of generative AI services over the past few months is that the generative AI can easily be used by anyone, unlike conventional AI, that requires highly specialist skills. Actlyzer is also characterized by its no-code UI operation. By combining pre-prepared basic movements with drag-and-drop, complex movement rules can be set, allowing non-technical personnel to customize the work movements they wish to recognize.
Shunichi: DeRA excels at accurately identifying anomalies. In a benchmark for anomaly detection in 2021, DeRA achieved the world's highest level of 98% in AUROC (Area Under the ROC Curve), an index that measures the performance of AI models in detecting anomalies (*9). Not only is HDL faster than other methods, it also achieves a lower error rate than conventional methods in an online learning benchmark of corrupted data as of 2022 (*10), as well as a level of accuracy equivalent or better than the state-of-the-art technology of the time.
Many customers using the AI Innovation Components have achieved business transformation
How are you utilizing the Operation Process Analysis component?
Atsunori: Fujitsu I-Network Systems Limited is actually using the Operation Process Analysis component. They are currently working on identifying priorities for improvement by using this component. For example, they can calculate unexpected work input or time spent not working by subtracting the actual work time from the allocated time. In addition, knowledge about the working time differences by operator and by product type represents important information for future improvement.
Customers have been using your technology from an early stage - have you discovered any conclusions that have led to further R&D?
Atsunori: Of course, there were essential features that we discovered only as a result of our customers using the system. For example, the need for worker identification and more detailed recognition of hand movements. We hope to solve our customers' problems while deepening our technology to realize such functions.
We have heard that the Product Defect Inspection component is also being demonstrated to customers.
Shunichi: We are currently inspecting vein sensor components at Fujitsu Frontech Limited as a demonstration test of this component. For vein sensor devices, we identify good ones and defective ones with scratches and stains. The objective is to achieve stable operation allowing for degradation of the AI model caused by environmental changes, especially during product inspection.
R&D to solve problems in the manufacturing industry
What technical challenges have you faced in the R&D of the Operation Process Analysis component? How did you overcome these challenges?
Atsunori: When we develop technology, we place great importance on ease of use and ease of implementation for our customers. In the early days of Actlyzer, we received comments from customers in the field that it was difficult for them to use the tool. The problem was that they could not operate it themselves, as the version being used was more or less the same as the researchers’ version used to perform the analysis. We have now made improvements and introduced a no-code interface, in which the customer specifies the combination of actions with a mouse operation, improving ease of use. We have also focused on overcoming another issue, involving differences in the environment of the customer's PCs at the site, making it difficult to install the system and to build the environment. We can now use the environment built in the cloud, which allows us to demonstrate the system quickly. For on-site demonstrations, we provide containers that can run on the customer's PC, making it easy to introduce the system to the site.
How do you approach the research problems?
Atsunori: I believe it is important to combine the top-down approach of narrowing down the functions and technologies needed based on customer requests with the bottom-up approach of applying cutting-edge technologies to components. Although cutting-edge technologies are being announced rapidly in AI research, there is a long delay before they are used by customers. I believe that Fujitsu Kozuchi can support this process of implementation by directly focusing on the functions required by the customer. I am also in discussion and jointly developing elements with many development members globally, such as in our research center in China and India. Thanks to teleworking, we are able to communicate with them regardless of location and time.
Some researchers have expressed a concern re the impact of their work on society. How do you feel about the balance between R&D and real-world problem solving in the field?
Shunichi: I believe that having customers try out Fujitsu Kozuchi is one very important way to encourage researchers to realize their technological contributions. Also, although it is not limited to Fujitsu Kozuchi components, I believe that the most important thing is for the solution to fit the problem that the customer really wants to solve, so I cherish every opportunity to talk with each customer.
The future of manufacturing applying AI as envisioned by both of them
What are your thoughts on the future prospects and social impact of the technologies you are currently researching and developing?
Atsunori: Changes in the environment surrounding the manufacturing industry are becoming more and more pronounced. AI is expected to provide solutions to issues such as the decrease, aging, and diversification of engineers, as well as responding to mass customization and to increasingly sophisticated after-sales support requests. Some may feel that the Operation Process Analysis component monitors workers, but I believe that this technology can protect workers and help create a more comfortable work environment in the rapidly changing manufacturing industry. Currently, some work contents are not yet fully covered, but I would like to expand its functionality as a component based on customer needs and the latest technologies. By accelerating the research and development of AI, I hope to contribute to making factory operation smarter throughout society.
Shunichi: With the recent rise of generative AI, the general public has become more familiar with the existence of AI. As evidenced by the surge in stock prices in the semiconductor sector, which is indispensable for AI development, this trend is beginning to boost the B-to-B AI business. As AI services become more widespread, I feel that the day is rapidly approaching when customers will face the next challenge of stable operation and quality improvement during operation. The HDL technology for Product Defect Inspection, will become indispensable technology for AI operation in the future. This technology is exactly what will be required when AI becomes a pillar of various social services, and I believe it will have a great impact.
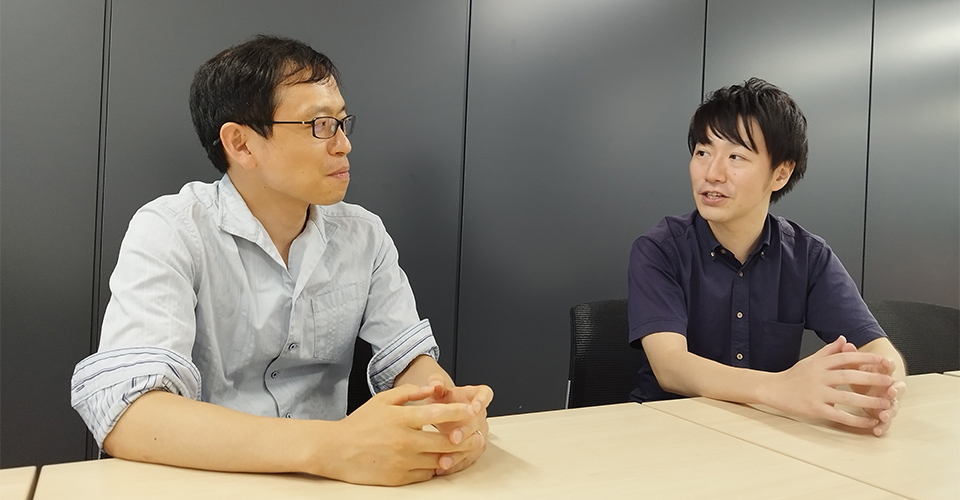
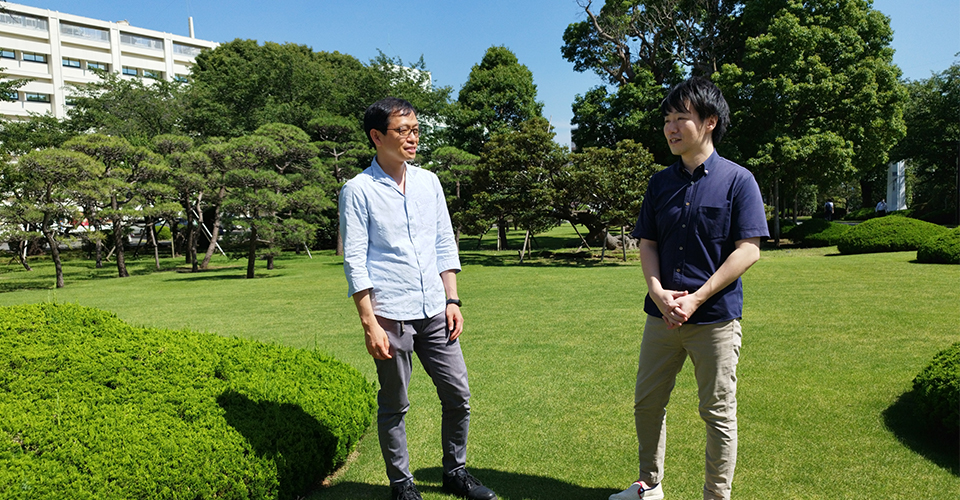
-
(*1)
-
(*2)
-
(*3)
-
(*4)
-
(*5)
-
(*6)ICPR (International Conference on Pattern Recognition) is an international conference on the field of pattern recognition.
-
(*7)ICIP (IEEE International Conference on Image Processing) is an international conference on image processing
-
(*8)ECCV (European Conference on Computer Vision) is an international conference on computer vision and AI
-
(*9)
-
(*10)
Notes (*4), (*10) only available in Japanese
Fujitsu's Commitment to the Sustainable Development Goals (SDGs)
The Sustainable Development Goals (SDGs) adopted by the United Nations in 2015 represent a set of common goals to be achieved worldwide by 2030. Fujitsu's purpose — “to make the world more sustainable by building trust in society through innovation”—is a promise to contribute to the vision of a better future empowered by the SDGs.
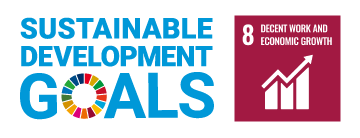