Content-Aware Computing Technology for Accelerating Increasingly Complex and Massive AI Processing
Release date: July 7, 2021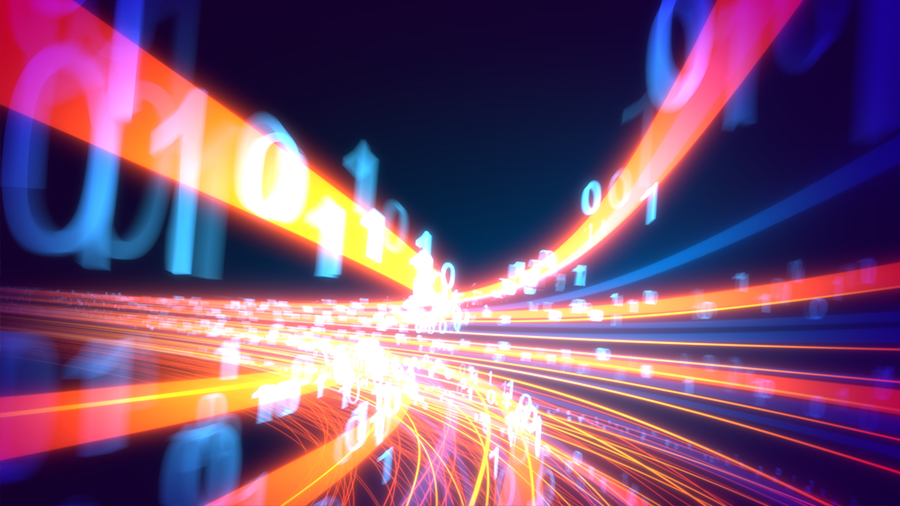
Fujitsu is conducting research and development on Content-Aware Computing (CAC) technology as a computing technology for meeting increasingly complex and massive computing needs. This technology increases processing speed by optimizing software based on analysis of processing content and data. By combining CAC technology, which consists of dynamic reduction of computational complexity, parallel training acceleration, and I/O acceleration, we achieved the world’s top processing speed on MLPerf HPC, a benchmark suite for large-scale machine learning processing, in November 2020. This article describes CAC technology and some application examples.
- 1. Introduction
- 2. Challenges toward accelerating AI processing
- 3. Content-Aware Computing technology for accelerating AI processing
- 4. Application examples
- 5. Summary and future work
1. Introduction
With the explosive growth of data driven by IoT and advances in data analysis technology through AI, a data-driven society that will utilize data as a new resource and transform society and industry is emerging. Computing power for training AI models, which continue to grow in complexity and massiveness, will become increasingly important for advanced analysis of huge amounts of data. For example, the text generation language model Generative Pre-trained Transformer 3 (GPT-3) has 175 billion parameters, and it is said to require 355 years to train on a single graphics processing unit (GPU) [1].
To meet these increasingly complex and massive computational needs, Fujitsu has been conducting research and development on Content-Aware Computing (CAC) technology [2]. CAC technology accelerates software processing based on analysis of processing content and data.
This article describes CAC technology for accelerating AI processing, which is becoming increasingly complex and massive, and some application examples.
2. Challenges toward accelerating AI processing
AI models are becoming increasingly complex and massive, and getting computers to rapidly train them requires computer hardware performance and software technology that can make the most of that performance. In this article, we will focus on deep learning, a type of AI processing that requires particularly large amounts of computation.
In deep learning, data such as images, sounds, and sentences are fed to a deep (i.e. multilayered) neural network (DNN), and by training the system tasks such as classification and regression, a model is acquired that recognizes images and sounds, translates sentences, and so on.
Deep learning requires enormous computational complexity but not high calculation accuracy, so it is important to reduce computational complexity through optimal control of calculation accuracy. On the other hand, in parallel computing using multiple computers at the same time, it is important to perform calculations with high efficiency while maintaining accuracy. Furthermore, it is important to reduce the time for copying huge amounts of data from the parallel file system to local disks and the delay time for reading from local disks to memory.
3. Content-Aware Computing technology for accelerating AI processing
CAC technology [3] is software technology that accelerates processing by analyzing processing content, reducing the processing amount, and optimizing the allocation of processing to computers. Figure 1 shows an outline of CAC. For a variety of applications, it is possible to speed up training by up to ten times by analyzing the processing content and, based on the analysis results, automatically executing optimization control of computational complexity, parallel processing, and I/O according to the hardware used.
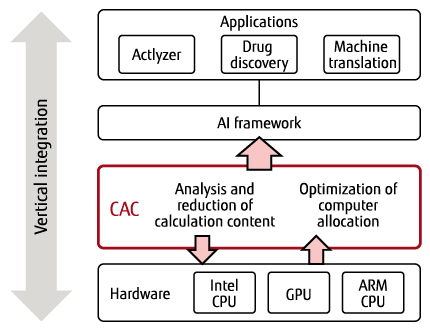
Figure 1 Outline of Content-Aware Computing.
In this section, CAC technology is described in the following order: Technology for dynamically reducing computational complexity, parallel training acceleration technology, and I/O acceleration technology.
3.1 Technology for dynamically reducing computational complexity
While deep learning requires enormous computational complexity, it does not require high computational accuracy, so optimally controlling computational accuracy to reduce computational complexity is effective for reducing training time. Figure 2 shows an outline of the technology for dynamically reducing computational complexity. Bit width reduction technology, Gradient Skip technology, pruning, and computational science simulation acceleration are introduced below as technologies that reduce computational complexity while maintaining learning accuracy.
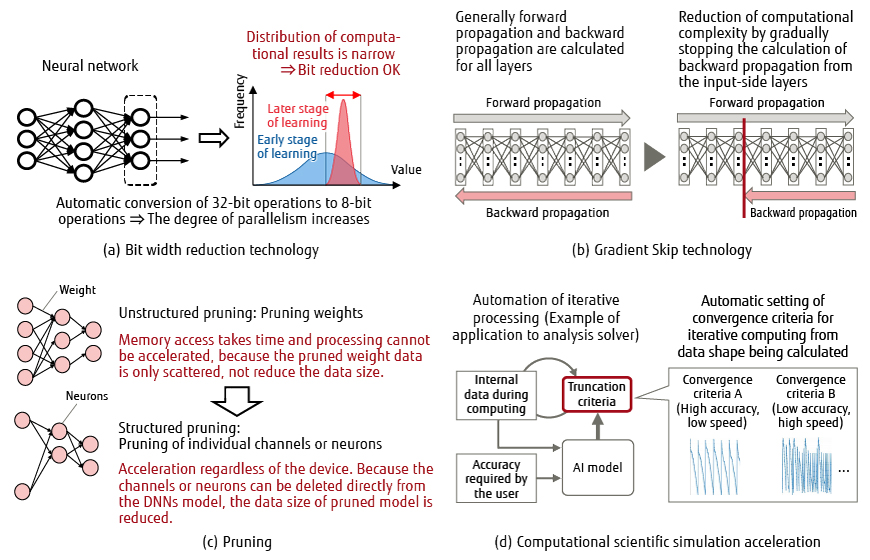
Figure 2 Technologies for dynamic reduction of computational complexity.
1) Bit width reduction technology
One of the methods for speeding up numerical calculations is bit width reduction technology {Figure 2 (a)}. It reduces data size by using 16 bits or 8 bits to represent numerical data, which is generally represented by 32 bits, to increase computation and communication speed.
In deep learning, the validation accuracy of DNN with reduced bit width may be remarkably degraded by careless bit width reduction. In the past, since bit width adjustment by an expert was required to prevent accuracy degradation, it was difficult to apply bit width reduction technology. To solve this problem, we have developed technology that automatically determines bit width that does not degrade accuracy, so that anyone can use bit width reduction technology. We applied ImageNet, a typical image classification task, to the training of AlexNet, ResNet-18, and ResNet-50, which are image processing DNNs, and they were able to automatically determine bit width without significant degradation in classification accuracy. Further, AlexNet and ResNet-18 achieved 3.5 times faster training, and ResNet-50 achieved 2.5 times faster training.
2) Gradient Skip technology
A DNN is a network that consists of multiple layers. There are two types of processing during training: forward propagation, which outputs inferential probabilities, and backward propagation, which calculates the amount of update (error gradient) for parameters (weights) in training. Weight update is not required in the layers where training has sufficiently advanced. Further, in image processing DNNs, it was found that parameters converge faster in input-side layers. For this reason, the Gradient Skip technology achieves higher speed by gradually stopping backward propagation computation starting from the input-side layers where training has progressed sufficiently, thereby reducing computational complexity {Figure 2 (b)}.
Having found from experiments that final training accuracy is slightly lower if weight update is stopped abruptly, we developed an accuracy assurance technology that smoothly reduces the update value to zero. As a result, accuracy degradation due to the application of the Gradient Skip technology was reduced to a negligible level. When we actually applied this technology to DeepCAM, which identifies extreme weather events from meteorological data (an MLPerf benchmark for HPC), a maximum speed increase of 1.8 times was achieved.
3) Pruning
The data size of DNNs continues to increase in order to achieve higher recognition accuracy and more complex pattern recognition. However, neural networks achieved by training include connections whose weight, which indicates the strength of the connections between neurons, can be regarded as zero. Because the results of operations (multiplication) for such connections can be regarded as zero, these operations can be omitted, thereby reducing computational complexity. Pruning technology [4] automatically identifies and deletes connections with small weight values in a DNN {Figure 2 (c)}.
A simple implementation would be to read the weights from memory and skip the operations that are considered to produce the value of zero. However, this simple implementation takes time to access the memory, and pruning cannot be used to increase speed. This is because the data whose operations should be skipped are only scattered, and the data size of the scattered data is not reduced. This time, we calculated the sum of the absolute values of the groups (channels) with the smallest weights, and deleted the channels with the smallest sums to directly reduce the data size of DNNs. Doing so ensured that unnecessary memory accesses would not be generated and that parallel processing could be utilized to achieve higher speed.
4) Computational scientific simulation acceleration
In computational science simulations such as structural analysis, fluid analysis, and molecular dynamics calculation, there is a growing need for large-scale calculations for obtaining detailed results, and for high-speed processing able to quickly simulate a large number of patterns for design automation and other applications.
In such calculations, it is important to set convergence criteria for efficiently achieving the accuracy required by the user in iterative calculations. In the past, the setting of convergence criteria was left to the user. This time, by using an AI model, we have developed a technology to judge the achievement of accuracy with minimal computational cost for the data being calculated {Figure 2 (d)} [5].
In addition, the use of surrogate models, which are obtained by training the relationships between inputs and outputs from data obtained in the process of simulation, is also making progress. By replacing simulation computations with a trained surrogate model, results can be obtained instantly.
3.2 Parallel training acceleration technology
In large-scale parallel training using a large number of computers simultaneously, it is important to increase the degree of parallelism while maintaining high efficiency. Figure 3 shows an outline of the parallel training acceleration technology. This sub-section looks at synchronization mitigation technology that maintains efficiency by reducing synchronization latency for processes that experience processing delays, and model-parallel training that achieves parallelism beyond the limits of data parallelism.
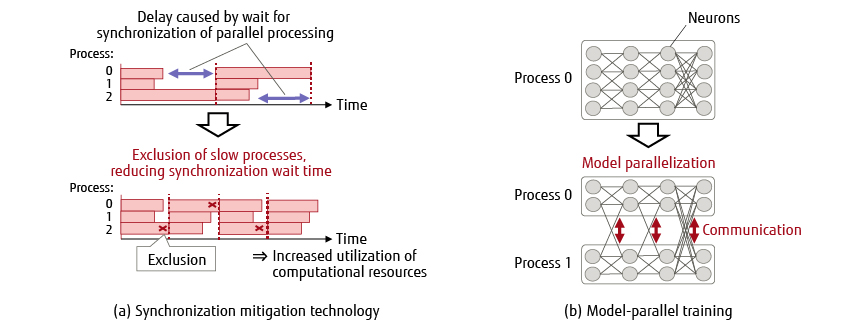
Figure 3 Parallel training acceleration technology.
1) Synchronization mitigation technology
Figure 3 (a) shows an outline of the synchronization mitigation technology. In distributed training for deep learning, the occurrence of processes with slow processing speed during training means that the entire processing will be delayed by the synchronization wait time required for each training iteration. To remedy this delay, we developed a technology that dynamically separates slow processes so that the processing speed is always maximized, thereby enabling training while suppressing slowdowns [6]. In ImageNet image classification using ResNet-50, we confirmed that the system can be trained with 25% process reduction with almost the same accuracy.
2) Model-parallel training
Figure 3 (b) shows an outline of model-parallel training. Model parallelism is a training method in which one DNN model is divided into multiple parts and distributed to computers for training. In addition to improving training speed, this approach enables training of a large-scale DNN model that is too big to be accommodated by a single computer. Data parallelism, in which multiple computers process different training data at the same time, increases the amount of data that can be processed at one time (batch size). As the batch size increases, the accuracy of the model obtained by training becomes lower. In the case of model parallelism, the batch size does not change, so the parallelism does not affect accuracy.
There are various model splitting methods for model parallelism. For example, the input data can be divided by spatial dimensions (height and width for images), or by channel (RGB for images). The type of communication between computers and the maximum number of parallelization depend on the input data, the DNN layers, and the splitting method, so it is necessary to select the splitting method that best suits the situation. The application of model parallelism requires expert knowledge. However, combined with data parallelism, it further accelerates training. We applied model parallelism to an MLPerf HPC benchmark described in Section 4 and achieved 1.8 times faster training speed with a 4-split model.
3.3 I/O acceleration technology
As deep learning uses a huge amount of data, I/O acceleration technology is required. This sub-section introduces the two techniques shown in Figure 4: data staging acceleration, which moves huge amounts of data from the parallel file system to local disks, and I/O bottleneck elimination, which conceals the time required to move data from local disks to memory during computing.
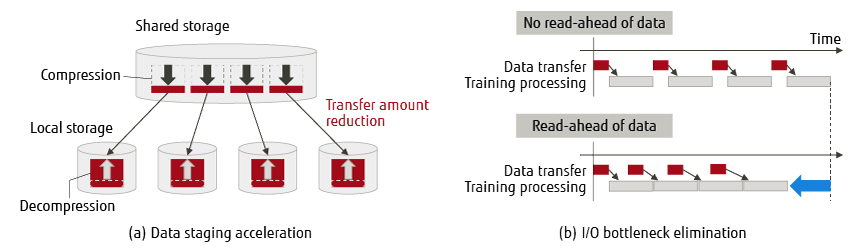
Figure 4 I/O acceleration technology.
1) Data staging acceleration
In training using a large number of computers, reading data from remote storage may cause conflicts and delays. This can be mitigated by using instead local storage with data staging. For even faster staging, it is effective not only to use high-performance storage and networks to increase throughput, but also to compress data to reduce the amount of data transferred. In particular, if training data can be compressed prior to storage, use of a high-compression format can be expected to significantly reduce data transfer time. Data is decompressed and transferred concurrently, and also staged across many computers to parallelize the decompression. As a result, the decompression time is reduced.
2) I/O bottleneck elimination
Training data that does not fit in memory is stored in storage such as local HDDs and SSDs, and is read into memory for training as needed. However, if reading of training data starts only when it is needed, training will be interrupted until data reading is completed. Therefore, we implemented reading of the next training data from storage ahead of time to allow efficient execution of training without interruption.
4. Application examples
This section describes examples of the application of CAC technology.
4.1 MLPerf HPC
MLPerf HPC is an MLPerf benchmark suite for HPC, and is widely used as a benchmark for machine learning [7]. It includes CosmoFlow, which estimates cosmological parameters from dark matter data, and DeepCAM, already mentioned in Section 3.1 2). Each benchmark measures training time, including staging. We have accelerated MLPerf HPC for the AI bridging cloud infrastructure (ABCI), of the National Institute of Advanced Industrial Science and Technology (AIST) and the supercomputer Fugaku of RIKEN [8].
For ABCI, staging is performed with both CosmoFlow and DeepCAM, and data compression is used for CosmoFlow. As a result, staging time was reduced to as little as 1/12. Furthermore, by eliminating the I/O bottleneck, training efficiency was improved by up to 20%. We also applied the Gradient Skip technology to DeepCAM to reduce training time by a further 10%.
For Fugaku, accelerated data staging and elimination of I/O bottlenecks were similarly implemented, but only for CosmoFlow. The performance per computer of Fugaku is lower than that of ABCI, but since Fugaku supports the use of more computers, the number of computers used was increased by up to 16 times, applying model parallelism in addition to data parallelism.
Through the application of CAC, ABCI achieved performance 20 times and 13 times higher in CosmoFlow and DeepCAM, respectively, compared to other GPU-type systems in MLPerf HPC v0.7, and Fugaku achieved performance 14 times higher in CosmoFlow compared to other CPU-type systems. As a result, ABCI and Fugaku achieved first and second place for large-scale machine learning processing in the world {Figure 5 (a)}.
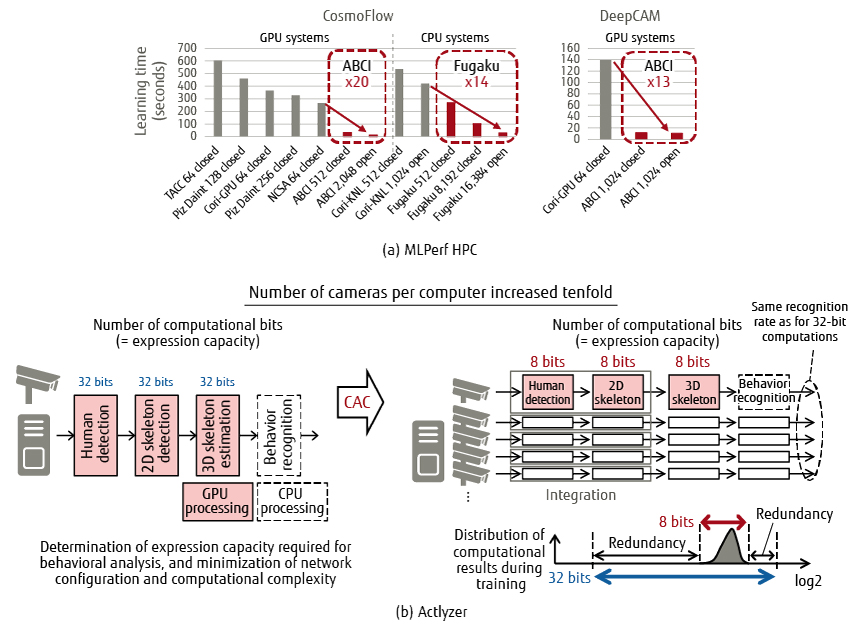
Figure 5 Application examples of CAC technology.
4.2 Actlyzer
Actlyzer is behavioral analysis technology developed by Fujitsu and Fujitsu R&D Center Co., Ltd. [9]. To increase the value of solutions that utilize this technology, it was necessary to increase the number of cameras that can be processed by one computer. To this end, computational waste in behavior analysis was identified and computational complexity was minimized by integrating human detection and skeleton detection, which are components of Actlyzer. Furthermore, the number of cameras that can be processed per computer was increased tenfold {Figure 5 (b)} by improving computation efficiency with bit width reduction technology for reduction from 32 bits to 8 bits and also by reducing the weight of the models for human and skeleton detection with pruning technology.
5. Summary and future work
This article introduced CAC technology for accelerating AI processing, which is becoming increasingly complex and massive, and some application examples.
By combining acceleration technologies consisting of technology for dynamic reduction of computational complexity, parallel training acceleration technology, and I/O acceleration technology, we achieved the world’s top processing speed on the MLPerf HPC benchmarks. Further, by applying Actlyzer, Fujitsu’s behavior analysis technology, we increased the number of cameras that can be processed per computer by a factor of ten.
Going forward, we will continue to perfect CAC technology, improve its speed and ease of use, and expand its application to various fields such as medicine, drug discovery, materials, and logistics, thereby contributing to the solution of social issues.
All company and products names mentioned herein are trademarks or registered trademarks of their respective owners.
References and Notes
About the Authors
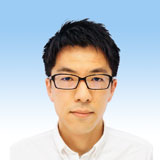
Mr. Shirahata is currently engaged in research for Content-Aware Computing.
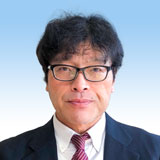
Mr. Hara is currently engaged in research for Content-Aware Computing.
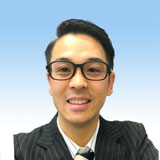
Mr. Sakai is currently engaged in research for Content-Aware Computing.
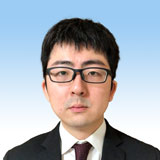
Mr. Miwa is currently engaged in research for Content-Aware Computing.
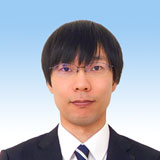
Mr. Tabuchi is currently engaged in research for Content-Aware Computing.
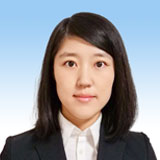
Ms. Gao is currently engaged in research for Content-Aware Computing.
Related Links
- Fujitsu, AIST, and RIKEN Achieve Unparalleled Speed on the MLPerf HPC Machine Learning Processing Benchmark Leveraging Leading Japanese Supercomputer Systems (Press Release)
- Content-Aware Computing: Automatically Adjusting Computational Accuracy to Accelerate AI Processing Tenfold (tutorial article)
- Fujitsu Develops New "Actlyzer" AI Technology for Video-Based Behavioral Analysis (Press Release)
- Fujitsu Develops Technology to Automatically Adjust Computing Accuracy to Accelerate AI Processing by 10 Fold (Press Release)