Researcher Interviews
Talking to the team behind Fujitsu Kozuchi (code name) - Fujitsu AI PlatformWorking to accelerate the social implementation of AI (Suspicious Behavior Detection chapter)
JapaneseSecurity cameras are a regular feature of our lives, with many public places installing them to safeguard their staff and customers, as well as to act as a deterrent for crime, terrorism, and suicide. However, it is prohibitively costly to have a large number of cameras constantly monitored in real-time, which impinges on their effectiveness for early detection and responses to accidents and crimes, or to preventing the spread of damage. Fujitsu has been working to solve these problems, and in this interview, we introduce the researcher Yoshiaki Ikai, who is in charge of the Suspicious Behavior Detection (*1) component that is included in the Fujitsu Kozuchi (code name) - Fujitsu AI Platform (*2). A component is a package of AI technologies needed to solve a problem. Yoshiaki tells us about how the Suspicious Behavior Detection component can enable rapid response to accidents and crimes, and he shares his overall thoughts on this important initiative.
Published on November 22, 2023
RESEARCHER
-
Yoshiaki Ikai
Senior Research Manager
AI Innovation Core Project
Artificial Intelligence Laboratory
Fujitsu Research
Fujitsu Limited
Initiatives for a safe and secure society
What prompted you to develop the Suspicious Behavior Detection component?
Yoshiaki:After the press release of Actlyzer (*3), a behavioral analysis technology developed by Fujitsu, we received many inquiries from customers. For example, they flagged up the serious social problem involving the rising number of train accidents. They wanted to know whether we could suggest a way of notifying station staff immediately after detecting signs of a potential accident, rather than having to check the history of camera images after an accident has occurred. We thought that if we could use images from existing security cameras to help prevent crimes and suicides at a realistic cost and with realistic personnel, we would be able to provide security and safety to many people. That was the impetus for the development of the component.
What challenges does the Suspicious Behavior Detection component solve?
Yoshiaki:The Suspicious Behavior Detection component utilizes Actlyzer to detect and analyze people and objects from video images and detect suspicious behavior. When a small number of people are monitoring a large number of security camera images, it is very easy to overlook something important. However, the Suspicious Behavior Detection component allows AI to detect suspicious behavior automatically, alerting security guards with an on-screen display, enabling a quick response when an incident or accident occurs.
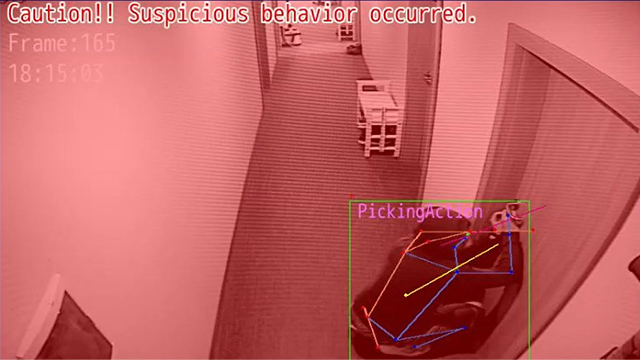
How are you utilizing the Suspicious Behavior Detection component?
Yoshiaki:Currently, we are actively conducting demonstration tests and introduction studies with several customers. As specific use cases in the public places, we are considering detecting suspicious behavior at airports and train stations where many people enter and exit. An example of this involves any detection of intrusions into restricted areas or of suspicious objects left unattended. We are also considering use cases such as the real-time detection of group theft at retail stores to prevent damage, as well as checking if young children are playing dangerously on playground equipment in parks. In this way, we hope to provide security and peace of mind to many people by introducing our Suspicious Behavior Detection component, whether in the retail industry and in places closely related to our daily lives.
A solution that overcomes conventional AI development issues and shortens development time
In the past, learning human behavior for video recognition AI required preparing a large number of videos that showed human behavior. Each behavior had to labeled with the correct answer, requiring several months’ development and significant cost. The Suspicious Behavior Detection component is equipped with Actlyzer, a behavior analysis technology developed by Fujitsu, which has pre-trained models of about 100 basic behaviors, such as "walking" and "raising hands". The combination of these basic behaviors enables the system to recognize a variety of behaviors, making it possible to develop AI in a short period of time, without spending time on the preparation of large amounts of training data and prior validation.
What technical challenges have you faced in the R&D of the Suspicious Behavior Detection component?
Yoshiaki:Behaviors such as a person entering a specific area or reaching for a shelf can be recognized with a combination of Actlyzer's basic movements. However, indefinite, subtle movements are much more difficult to recognize. Suspicious behavior is often less obvious than normal behavior, such as looking furtively around or peering into spaces, so it was difficult to distinguish suspicious behavior from normal behavior with the combination of basic movements. In order to recognize such subtle movements, the usual development process involves collecting a large amount of new data and having the AI learn the behavior, but this is time-consuming and costly.
How did you overcome these challenges?
Yoshiaki:For actions that we had difficulty recognizing in the basic behavior of the Actlyzer, we have developed a technology incorporating few-shot learning (*4) to add new behaviors that can be recognized by Actlyzer. Based on a small amount of video data, the system can efficiently learn any suspicious behaviors to be recognized and can handle special cases. Thanks to this approach, we are now able to handle more use cases, maintaining the component’s key advantage of being able to capture even subtle behaviors without sacrificing the ease of implementation.
How do you approach solving the research problems?
Yoshiaki:We place great importance on listening to our customers and reflecting their feedback in our development technologies. To accomplish this, we adopt a four-step approach. First, based on Fujitsu’s business situation and the development team’s technical capabilities, we create a hypothesis that “if we create this kind of new technology, it will become a business”. Next, we develop a prototype for demonstration. We then work with sales and business divisions to introduce the technology to customers and conduct demonstration tests, receiving feedback from customers. Based on the feedback, we then improve the prototype. Only by repeating the process of improving prototypes based on customer feedback can we create new technologies that can be applied at the customer's site. In addition, new technologies developed in this process can be evaluated objectively using home-grown data and open data, and will be presented at conferences and in papers.
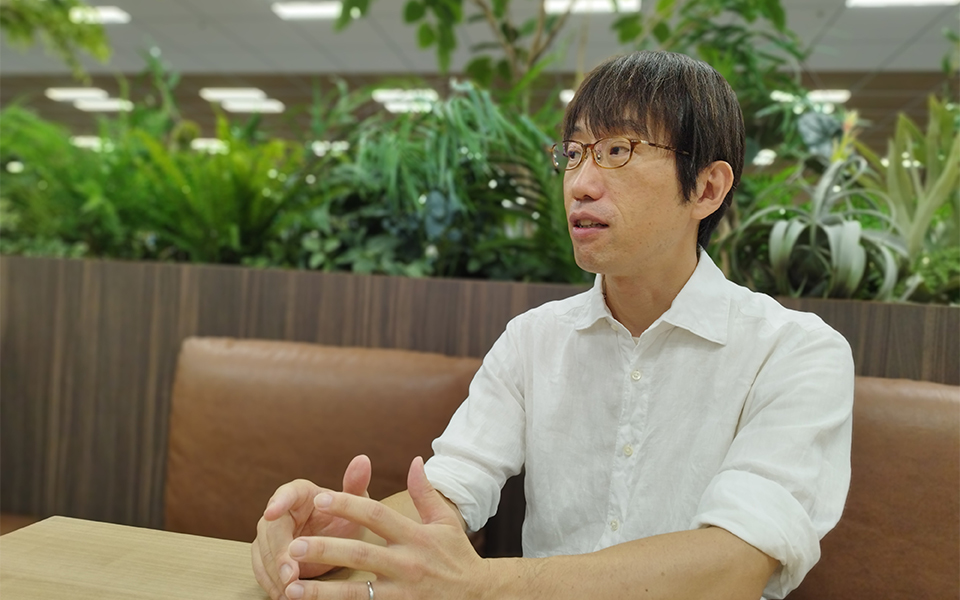
The future envisioned by researcher supporting customers' AI innovations
How do you balance R&D with solving problems in the field?
Yoshiaki:Usually, my first priority is to make commitments to customers, such as demonstration tests and software improvements. I believe that activities to solve problems in the field are important. On the other hand, R&D is also carried out quickly and efficiently based on feedback from our customers. We, the development team of the Artificial Intelligence Laboratory, are like a venture company, and we are constantly having our customers try out our cutting-edge technologies. It is challenging, but I find it rewarding to improve our technology by reflecting customer feedback.
What are your future ambitions for achieving a safe and secure society?
Yoshiaki:Unfortunately, violent incidents in public places, suicides on trains, and thefts in stores occur on a daily basis. While it is very important to investigate incidents after they occur, it is far better for everyone if we can prevent them before they happen. The Suspicious Behavior Detection component is a technology that can be used to prevent incidents before they happen as well as to respond quickly to accidents and incidents when they do occur. There is still room for further improvement, such as introducing further cost reductions and improved recognition accuracy. However, we intend gradually to improve the technology, taking into consideration important legal and ethical aspects such as profiling and privacy, as well as introducing advanced crime prevention systems in more places to realize a safer and more secure world.
-
(*1)
-
(*2)
-
(*3)
-
(*4)Few-shot learning is a technique that efficiently performs machine learning with much less data than is normally required for training.
Fujitsu's Commitment to the Sustainable Development Goals (SDGs)
The Sustainable Development Goals (SDGs) adopted by the United Nations in 2015 represent a set of common goals to be achieved worldwide by 2030. Fujitsu's purpose — “to make the world more sustainable by building trust in society through innovation”—is a promise to contribute to the vision of a better future empowered by the SDGs.
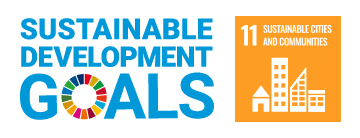