Researcher Interviews
Talking to the team behind Fujitsu Kozuchi (code name) - Fujitsu AI PlatformWorking to accelerate the social implementation of AI (Consumer Behavior Analysis chapter)
JapaneseThe retail industry faces many challenges with regard to understanding changing consumer needs, a lack of manpower and experience, and other issues such as POS information alone not telling us why a consumer decides to purchase a product or not and as a result, creating challenges for improving store layouts due to the lack of any real data to base decisions on. To solve these issues, Fujitsu has announced a new AI Innovation Component that supports the realization of smart stores. It does this by providing detailed visibility into purchasing behavior in real stores, which until now has been a black box, in order to deliver a personalized customer experience.
We recently interviewed the researcher in charge of the Consumer Behavior Analysis (*1) component – a package of AI technologies needed to solve a problem - for the retail industry that will be included in the Fujitsu Kozuchi (code name) - Fujitsu AI Platform (*2), involving. His insights detail how the Consumer Behavior Analysis component, which utilizes Actlyzer (*3), a behavior analysis technology that detects consumer behavior in real stores with high accuracy, can improve the consumer purchasing experience and increase operational efficiency.
Published on October 24, 2023
RESEARCHER
-
Shun Takeuchi
Senior Research Manager
Human Reasoning Core Project
Artificial Intelligence Laboratory
Fujitsu Research
Fujitsu Limited
Solving store operation issues by using data to achieve transformation
How did you approach the smart store concept, and what did you do to promote the demonstration experiment with your customers?
Shun:In recent years, real stores have shifted to somewhere that provides a genuine customer experience. The strength of real stores is that they can actually identify the demographics of visitors and whether they came alone or with their families. Many managers believe that it is important to visualize in detail the behavior of customers and utilize this information in store operations. However, due to a lack of manpower on the retail front, consumer behavior analysis has not progressed as far as it could. So our ambition is to help improve the customer experience by providing a Consumer Behavior Analysis component to clarify visitors’ in-store behavior, leading directly to in-store improvements.
How are you utilizing the Consumer Behavior Analysis component?
Shun:The Consumer Behavior Analysis component recognizes and analyzes people and objects from video images by making full use of the behavioral analysis technology Actlyzer. This technology, enables the detailed digitization of purchasing behavior in real stores, which is not possible with POS data. The current shortage of store clerks in the retail industry is becoming more serious than ever in recent years, and we considered the possibility of using AI technology to operate stores more efficiently without increasing the number of store clerks. The Consumer Behavior Analysis component can address this issue in two ways. The first is to detect the distinctive purchasing behavior of visitors to the store and allow store staff to serve them effectively in that location. Depending on the time of their visit and the degree of congestion, store clerks can be assigned appropriately. The second is that stores can be created based on visitor behavior data. Until now, product displays have been based solely on the intuition and experience of the person in charge. The Consumer Behavior Analysis component can be used to improve shelf allocation by visualizing the reach-out rate and the distribution of where visitors reach for products on the shelves, which can be used to improve sales.
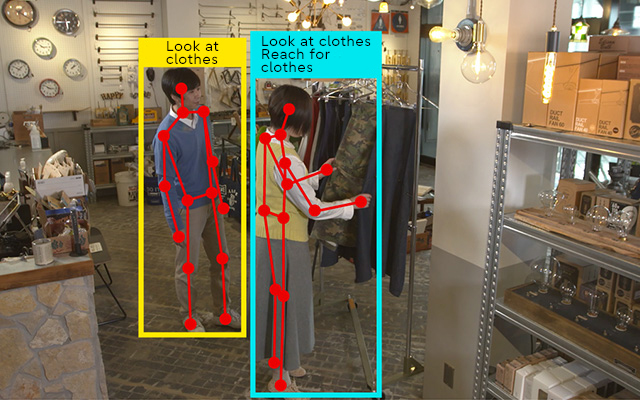
How are you utilizing the Consumer Behavior Analysis component?
Shun:Demonstration tests are underway in actual stores, including supermarkets and unmanned stores (*4). The Consumer Behavior Analysis component detects any product shelves where visitors spend the most time and their behavior with respect to comparing multiple products. Based on this information, digital signage installed in the store is used automatically to recommend products. In this way, we are conducting demonstration experiments with many customers using the Consumer Behavior Analysis component to support on-site activities such as sales promotion effects.
Promoting R&D that matches customer needs with technology seeds
Shun:Fujitsu believes that by combining high-precision technology with knowledge from the humanities and social sciences, such as behavioral science and psychology, we can contribute to solving complex issues in society. Actlyzer, the behavior analysis technology incorporated in the Consumer Behavior Analysis component, is capable of recognizing human behavior from camera images. By using a model that has learned about 100 basic behaviors, it can recognize various human behaviors from video without spending time preparing large amounts of training data or conducting preliminary verification. It is currently used in various areas such as marketing and security.
What was the impetus for the development of the Consumer Behavior Analysis component?
Shun:A common way to understand consumers' needs is to survey them to find out what kind of products they are interested in. Also, by analyzing what visitors bought at a store with POS data, we can find hints for improving sales and managing the number of items in stock. However, this does not tell us what products visitors did not buy or what products they actually picked up and compared. We thought that deeper knowledge of this would be useful for efficient store management, which led to the development of the component.
What technical challenges have you faced in the R&D of the Consumer Behavior Analysis component?
Shun:It is rare for a single camera to capture the entire sequence of a customer's activities from entering to leaving a store. Person Re-identification (ReID), a technology to identify the same person across multiple cameras, is one of the key elemental technologies for analyzing the details of purchasing behavior. With the evolution of deep learning technology and the availability of large datasets for training, it has become possible to learn person features more effectively. Thus, ReID performance is improving year by year. However, it is known that performance degrades for domains that differ from the training data (e.g., different stores or different lighting brightness). This is called a domain gap; ReID datasets are more expensive to collect than typical image datasets, making domain gaps a major technical challenge.
How did you overcome these challenges?
Shun:To remedy this issue, we devised a new technology for domain-adaptive learning (UDA: Unsupervised Domain-adaptation), using data without supervised labels. The key point of the developed technology is that by utilizing multiple camera images in a store, it becomes unnecessary to collect label data for learning. In the analysis of purchasing behavior in real stores, a large number of cameras are installed to track the circulation behavior of customers. In such a situation, there will be overlapping areas where multiple cameras are shooting. We found that image data of the same person can be automatically obtained from these overlapping areas, and that a highly accurate UDA technique can be constructed by combining a mathematical model with neural network training. This technique can learn the best person features for the target store without manually collecting training labels, thus solving the domain gap issue. Benchmark tests, using both public and independently acquired datasets, confirmed that the proposed method achieves the best performance (SOTA). Our paper on this achievement was accepted for oral presentation at IEEE ICIP 2022, the top conference in the field of AI image processing (*5). We plan to implement this technology in future components.
How do you approach the research problems?
Shun:We value co-creation with other organizations. We promote demonstration experiments in close collaboration with business divisions and customers, as well as joint research with universities. On-site practice enables us to identify technical issues. In addition, clues to their solutions are often found through practice. By engaging in discussions with people from different industries, including business divisions, retail and manufacturing companies and academia, we feel that we can organically link theory and practice and create solid value. The Consumer Behavior Analysis component we have developed this time is truly the result of matching the needs of our customers with the seeds of our technology.
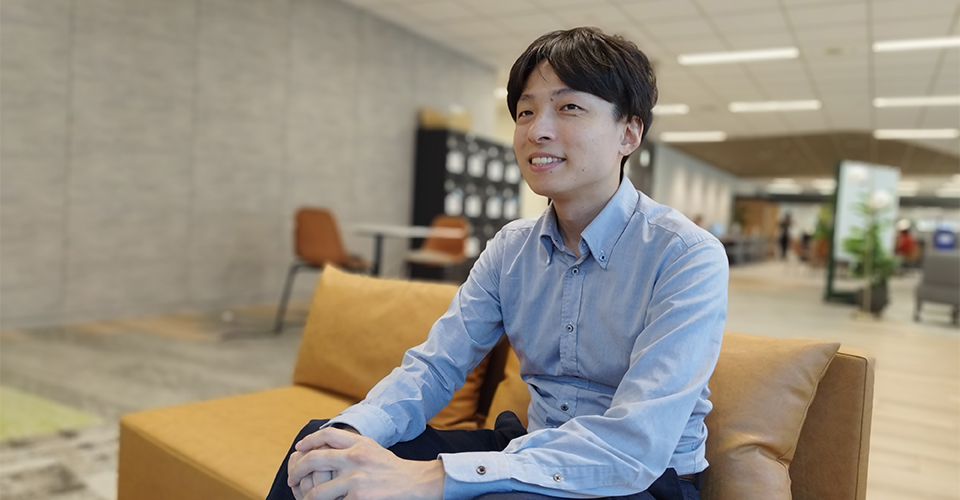
Aiming to enhance customer experience value, creating attractive and sustainable smart stores
Please tell us about your R&D toward the realization of smart stores and your outlook for the future.
Shun:Real stores are being rediscovered as places where consumer behavior can be analyzed in detail, and the nature of stores is changing. In recent years, the concept of stores that do not sell (*6) have merged and RaaS (*7) offerings have increased. I believe that the Consumer Behavior Analysis component can contribute to improving the value of the experience by visualizing the in-store behavior of visitors and providing insights. In addition, in recent years, it has become easier to generate content for marketing initiatives using generative AI, further expanding the scope of AI utilization. We are also taking on the challenge of analyzing consumer psychology by integrating humanities and social sciences, and research in behavioral analysis is entering a new paradigm. We will continue to develop advanced AI technologies and implement AI in society to realize attractive and sustainable smart stores.
-
(*1)
-
(*2)
-
(*3)
-
(*4)
-
(*5)
-
(*6)Stores that do not sell refers to a new form of retailing in which the purpose is not to sell products in stores, but only to try out and experience products, and to have customers use e-commerce sites when making purchases.
-
(*7)RaaS (Retail as a Service) refers to the combination of knowledge, such as data and know-how related to retail and retail business, and IT technology to provide it as a service.
Fujitsu's Commitment to the Sustainable Development Goals (SDGs)
The Sustainable Development Goals (SDGs) adopted by the United Nations in 2015 represent a set of common goals to be achieved worldwide by 2030. Fujitsu's purpose — “to make the world more sustainable by building trust in society through innovation”—is a promise to contribute to the vision of a better future empowered by the SDGs.
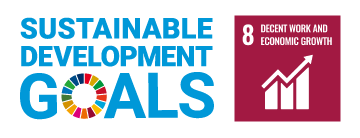