Fujitsu Research of America
Quantum Lab

Transforming society in the digital age with the world's most advanced computing
Our mission is to push the boundaries of cutting-edge computing technologies,
surpassing the limitations of Moore's Law and Von Neumann bottlenecks.
Quantum computing is a dynamic field of research and development that, when fully realized, will undoubtedly revolutionize the world of computing and society at large. Addressing some of the world’s most pressing challenges will necessitate scientific and technological innovations, where computing will play a crucial role. The quantum computing paradigm introduces a novel approach to processing information through quantum particles, offering the potential to alleviate the computational bottlenecks faced by several complex societal problems.
At Fujitsu Research of America, we delve into the capabilities and constraints of quantum computing, focusing on algorithmic development and applications. Our exploration spans various societal domains, considering both near-term and long-term perspectives. Actively researching the benefits of quantum algorithms for fundamental computational problems, we address specific issues and offer quantum solutions to enhance the resolution of our customers' challenges. Identifying quantum computing advantages across different computational models, applications, and industrial verticals is a crucial step in exploring the future usability of our research.
Researchers in the Quantum Lab
Jesus Gumaro Rendon Suzuki
Quantum algorithms,
quantum optimal control,
quantum many-body physicsAnkit Kulshrestha
Quantum algorithms,
Deep learning and Optimization algorithmsBibhas Adhikari
Quantum neural networks,
quantum linear algebra,
complex networksHannes Leipold
Quantum machine learning,
quantum annealing,
quantum algorithms
Hayato Ushijima
Quantum optimization,
quantum machine learning,
quantum algorithms,
quantum error mitigationRutuja Kshirsagar
Quantum error-correction,
quantum algorithms,
quantum machine learningSarvagya Upadhyay
Quantum information, complexity theory,
optimization, machine learning, differential privacyXiaoyuan Liu
Quantum optimization,
quantum machine learning,
quantum algorithms
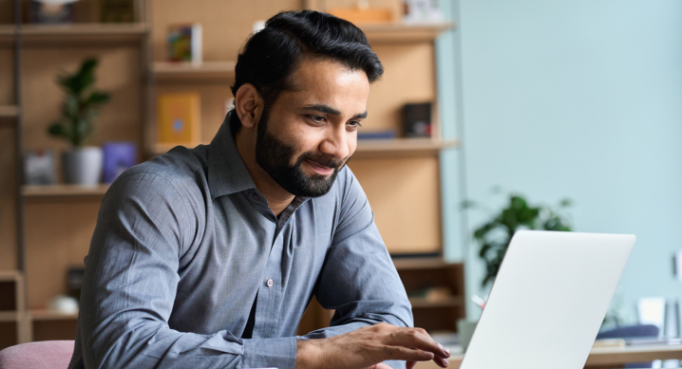
Publications
- Rohit Sarma Sarkar, and Bibhas Adhikari. Scalable quantum circuits for n-qubit unitary matrices, In Proceedings of the IEEE International Conference on Quantum Computing and Engineering, (IEEE QCE23), 2023.
- Rima Hazra, Mayank Singh, Pawan Goyal, Bibhas Adhikari, and Animesh Mukherjee. Modeling interdisciplinary interactions among Physics, Mathematics & Computer Science. To appear in the Journal of Physics: Complexity, 2023.
- Amrita Mandal, Rohit Sarma Sarkar, and Bibhas Adhikari. Localization of two-dimensional quantum walks defined by generalized Grover coins. Journal of Physics A: Mathematical and Theoretical, 56(2), p.025303, 2023.
- Bibhas Adhikari, Amrik Singh, and Sandeep Kumar Yadav. Corona product of signed graphs and its application to modeling signed networks, Discrete Mathematics, Algorithms and Applications, 15(1), 2250062, 2023.
- Alexey Galda, Eesh Gupta, Jose Falla, Xiaoyuan Liu, Danylo Lykov, Yuri Alexeev, and Ilya Safro. Similarity-Based Parameter Transferability in the Quantum Approximate Optimization Algorithm, Frontiers of Quantum Science and Technology, 2023.
- Dylan Herman, Cody Googin, Xiaoyuan Liu, Yue Sun, Alexey Galda, Ilya Safro, Marco Pistoia, and Yuri Alexeev. Quantum computing for finance, Nature Reviews Physics: 1-16, 2023.
- Krish Matta, Xiaoyuan Liu, and Ilya Safro. Decomposition Based Refinement for the Network Interdiction Problem, In Proceedings of the IEEE High Performance Extreme Computing Conference (HPEC), 2023.
- Anthony Angone, Xiaoyuan Liu, Ruslan Shaydulin, and Ilya Safro. Hybrid Quantum Classical Multilevel Approach for Maximum Cuts on Graphs, In Proceedings of the IEEE High Performance Extreme Computing Conference (HPEC), 2023.
- Ankit Kulshrestha, Xiaoyuan Liu, Hayato Ushijima-Mwesigwa, and Ilya Safro. Learning To Optimize Quantum Neural Networks Without Gradients, In Proceedings of the IEEE International Conference on Quantum Computing and Engineering (QCE), 2023.
- Monika Henzinger, Jalaj Upadhyay, and Sarvagya Upadhyay. Almost tight error bounds on differentially private continual counting. In Proceedings of the Annual ACM-SIAM Symposium on Discrete Algorithms (SODA), pages 5009-5039, 2023.