Researcher Interviews
Research for AI innovation – Fujitsu’s exciting new Indian center is set to transform AI research
JapaneseAt the heart of Fujitsu Research of India Private Limited’s activity (FRIPL) (*1) is the mission to focus on leading edge AI and quantum software, providing essential future software technologies to underpin R&D. India represents the perfect location for this focus, based on the incredible pace of IT growth and progress in recent years. FRIPL will accelerate joint research with top class Indian research institutes, such as the Indian Institute of Technology Hyderabad (IIT Hyderabad) and the Indian Institute of Science (IISc). Our joint goals are to contribute to resolving some of the world’s most challenging social issues through cutting-edge technologies and innovations. We invited four key members of our international AI research team from India, the UK and Japan to talk about their research and how it is making a valuable contribution to global sustainability.
Published on March 13, 2023
MEMBERS
-
Abhishek Chikane
Researcher
Autonomous Learning Project
Artificial Intelligence Laboratory
Fujitsu Research of India Private Limited -
Arkadipta De
Researcher
Autonomous Learning Project
Artificial Intelligence Laboratory
Fujitsu Research of India Private Limited -
Serban Georgescu
Research Manager
Autonomous Learning Project
Artificial Intelligence Laboratory
Fujitsu Research of Europe -
Hiromichi Kobashi
Project Director
Autonomous Learning Project
Artificial Intelligence Laboratory
Research Unit
Fujitsu Limited
Introducing our new AI talent from FRIPL
Fujitsu’s new team members Arkadipta De and Abhishek Chikane outline their project focus
Arkadipta completed his Master of Technology from IIT Hyderabad in Artificial Intelligence and Machine Learning, and is currently working on two projects, as he explains: “My first project has a complex-sounding title but is initially all about finding a better way of modelling post flood damage assessments. I am looking at ways of improving the Out-of-Distribution (OOD) generalization performance of Visual Question Answering (VQA). VQA involves giving a system a text-based question about an image, to which it has to infer the answer. Implementing flood countermeasures is a very time-consuming process. Let’s take the case of people stranded in a flooded area - the rescue team has to assess how many people are there, maybe take immediate action to evacuate them, or prepare emergency supplies to be dropped. But before they can do any of this, we first need to perform a thorough assessment of the damage so that the rescue can be planned based on accurate information. This is currently a real challenge, and we hope to improve it with VQA. My second project involves research on domain adaptation techniques on non-euclidean data such as point clouds. This is all about developing a technique to make the AI system understand and recognize a particular object in general, irrespective of any variation, appearance or the source of information capture. You can compare it to being able to recognize a car as a car, irrespective of its color, shape or type.”
Abhishek completed his masters from the Indian Institute of Technology Bombay (IIT Bombay), conducting his research at the Machine Learning and Visual Computing Lab. In addition to working on the same project as Arkadipta on flood detection with OOD generalization, he is also involved in performing physics simulations using AI, explaining: “it’s an exciting project aiming to use AI to build more efficient vehicles and aircraft. We are mainly focusing on structure simulations that enable us to save cost and reduce the time required on product manufacturing. For example, in traditional methods to produce a product, crash simulations such as drop tests and hit tests are costly and take time to complete. Physics simulations are expected to improve on both counts.
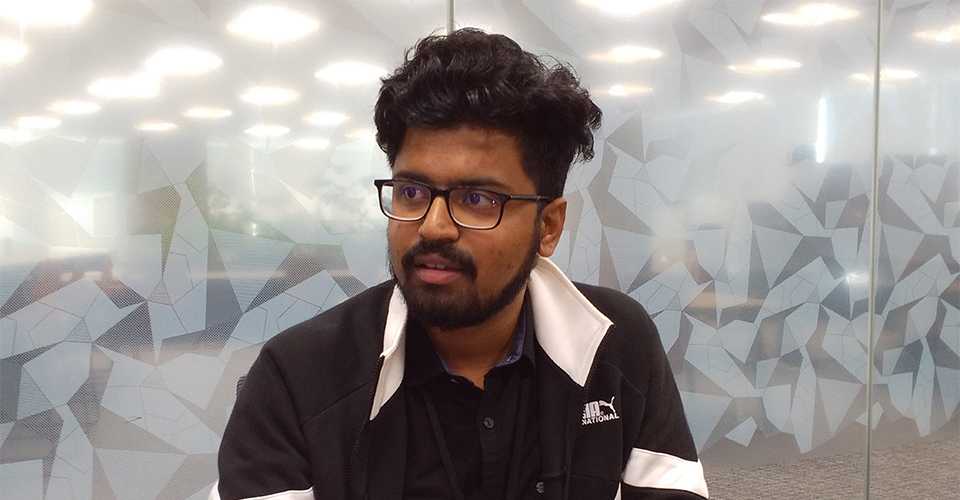
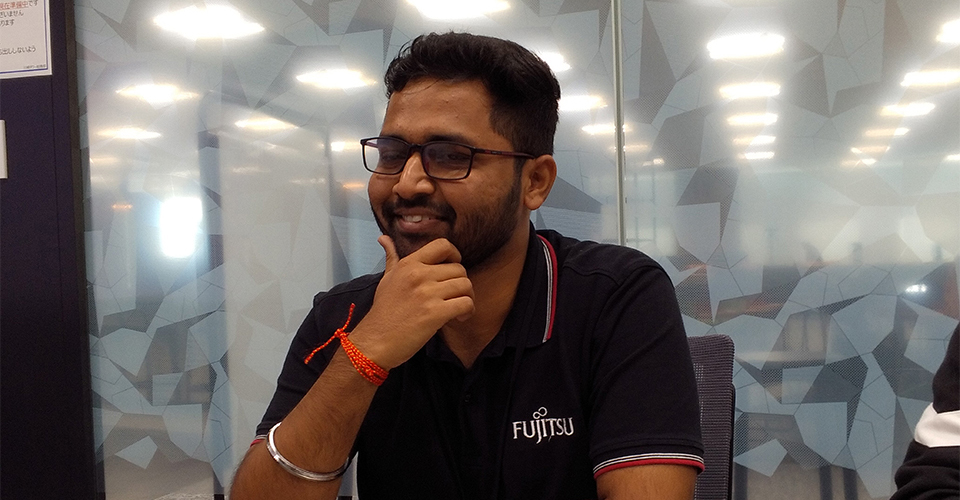
Pathway to Fujitsu
Arkadipta was aware of Fujitsu’s position as Japan’s largest leading IT Service provider with a significant world profile. He recalls: “Fujitsu also grabbed a lot of attention when they introduced Fugaku as the fastest supercomputer in the world, and that really made me interested in joining the company. Coincidentally, one of my PhD seniors, who graduated from IIT Hyderabad, is closely associated with Fujitsu Research of Europe (FRE) (*2) and that is how I first heard about and became interested in Fujitsu Research. But above all, Fujitsu Research offered me the opportunity to focus on exactly what I wanted to do, namely undertaking significant research in the field of AI.”
Abhishek was familiar with Fujitsu’s world's fastest computer Fugaku and their products such as computers and laptops. His route to his present position also involved a personal element: “A senior at my university worked in Fujitsu Research and he shared his research experiences in the company with me, giving me a good insight. I joined Fujitsu mainly because of their research work. I loved the fact that Fujitsu has shown deep involvement in research and development over the years.”
Initial impressions
It’s been a great start for Arkadipta: “After joining, I felt straight away that I have come to the right place and I am doing what I love the most, research work. It is an amazing place to work and grow. We are currently focusing on both theoretical and application research. In university, I never had a chance to conduct research from an application point of view, and I am finding that the hands-on-experience in industry level research projects gives me insights into my research from a very different perspective. The theoretical research we are conducting from a technical point of view is also accelerating my curiosity to think and solve problems.”
And for Abhishek as well: “The team conducts high quality research. But I have also come to realize that Fujitsu takes good care of their employees. The work life balance is good and at Fujitsu, people are very helpful. They motivate each other to be more creative and innovative. Also, we have a fantastic work environment. I personally love to visit the office frequently, even when we are allowed to have a hybrid work style and can work from home.”
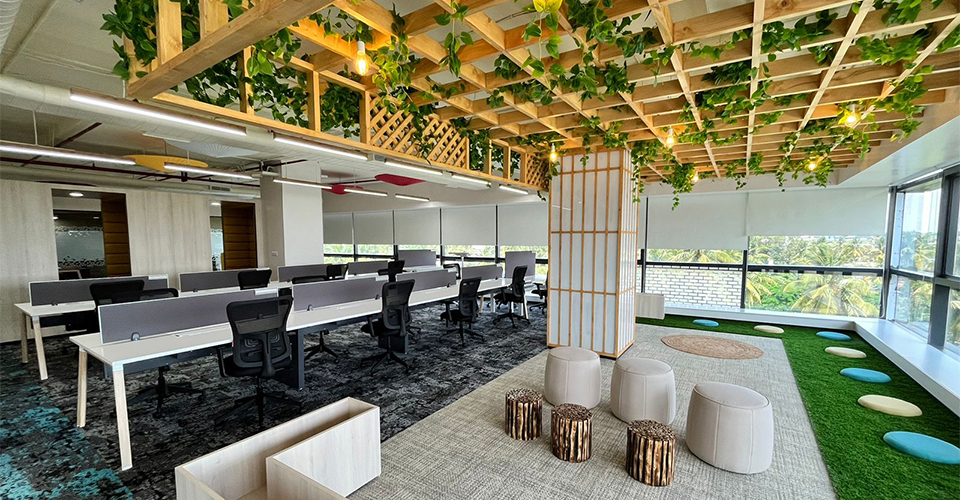
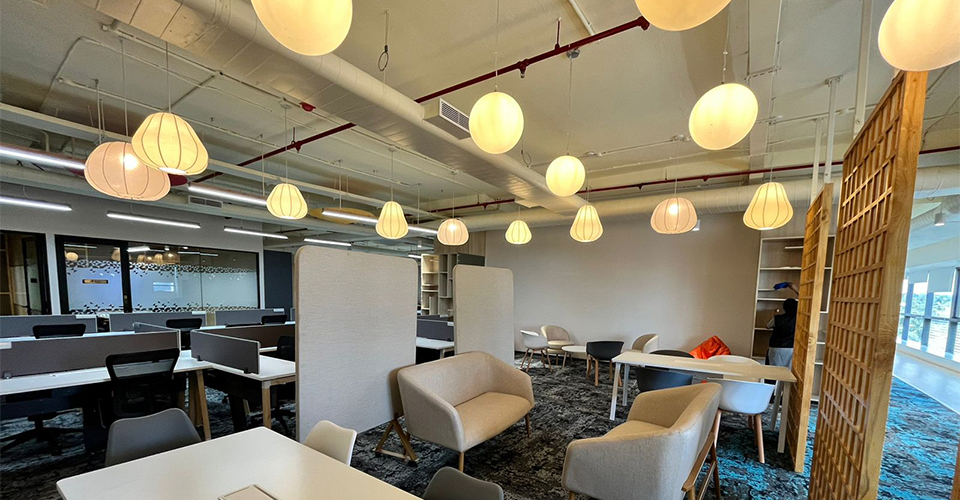
FRIPL new office. An open working space for workers.
Research focus on Out-of-Distribution generalization, with FRE and FRIPL working together on different social challenges
The primary target for the AI research project
Serban Georgescu is the research topic team leader, which focuses on understanding how Deep Neural Networks behave in OOD scenarios and findings ways to improve their generalization performance in different situations. He explains:
“The OOD research is being conducted jointly by our European- Indian- and Japan-based research teams, FRE, FRIPL and Fujitsu Research, and our target is to find applications relevant ideally for the UK, Japan and India. We want to create technology that can solve multiple applications as well as being related to businesses. In our team we have two starting points. One starting point is the technology point of view, which involves preparing models and techniques on academic data sets, and then looking for business opportunities. While it may not ultimately match the business needs, this prepares us to understand technological challenges. The second starting point is the application point of view, where we start from clear application needs and work towards finding a technological solution. While this approach may not ultimately result in new technology, it helps us understand the business needs and requirements much better. The end goal is hopefully to meet in the middle of what technology we need and how to match it to business!”
Research challenges
While AI has surpassed people in a number of areas, from games to medical scan interpretation, it is less widely known that this super-human accuracy drops very rapidly as one moves away from the training data. This is one of the major challenges with current deep learning systems, and is hindering its adoption in high stake fields such as medical diagnostics.
Serban takes up the theme with an example: “One amusing example that can be given to illustrate this is that of a pink elephant - an AI trained in the "classical" way cannot recognize a pink elephant, because it has never seen one in the training data. It would most likely say it is a flamingo. Considering the above, the goal of our research is to understand why the network cannot generalize well and to come up with new techniques that can overcome such limitations.
Going back to the pink elephant example, a neural network architecture that learns the concept of shape and color separately, say in different modules, can be successful at this task, since it will be able successfully to recognize the shape of an elephant without being confused by the color. Equally, it can recognize the color pink without considering the shape. By freely combining these two concepts, the challenge can be overcome. However, the biggest challenge in such research is that the concept of OOD generalization itself is very difficult to define. In essence, we want a system to generalize to what it has not seen before, but the number of possibilities is infinite. As such, one needs to be very careful in formulating hypothesis, creating datasets and performing experiments.
A pink elephant.
We have also extended our collaborative work more widely, working with the Massachusetts Institute of Technology ’s (MIT) to come up with improved AI model accuracy for OOD generalization (*3). MIT is a very academic team. In general, they take simple cases like colored letters or numbers, which are good enough to understand if certain mechanisms work, like why generalizations happen. Fujitsu’s role is more focused on applications, looking at how a simple concept can actually be applied in the complex real world.”
Applying Fujitsu's OOD generalization to the real world
Fujitsu’s OOD generalization research is already being applied to a variety of areas, as Serban explains: “We are initially looking into medical applications, aiming to increase the accuracy of computer vision models for example. The problem is that it drops dramatically when using data from a different scanning device to the one used for training data acquisition. We have also started looking into flood detection applications, where we need to make sure that the AI used can work in very challenging weather conditions where it is most needed. This is the project that Arkadipta and Abhishek recently joined, focusing specifically on the topic of flood detection.”
Focusing on flood detection
Arkadipta elaborates on India’s specific challenges in tackling floods: “In specific parts of India, for example in Assam, Jharkhand, some parts of West Bengal, and Mumbai, floods and waterlogging are really serious concerns. After a flood occurs in India, the Indian Military and disaster management groups are deployed in order to evaluate the damage and aid the affected people. One of the prime goals of our research is to improve the OOD generalization performance of VQA for flood damage assessments. By enabling both the government and disaster management groups to make better informed decisions, using a system where minimal manual intervention is needed in order to assess post flood damage, they will be able to get aid to everyone affected faster and more efficiently. That’s the goal!”
Serban takes up the theme: “There are three other key possibilities for OOD generalization contributing to the more effective handling of flood issues. Firstly, pre-flood activity can be detected earlier, for example 2 or 3 days in advance, enabling mass evacuations of people in potential danger. This type of research usually involves a combination of modeling and satellite imagery, mostly based on physical modeling.
The second example is still pre-flood but involves unpredictable phenomena that happen some hours earlier like flash floods. The detection of flash floods is mostly sensor-driven, involving for example, cameras located in important locations such as close to rivers or where flooding is expected to happen, but also in locations where it is not expected, such as in the city center. By observing the flood situation, warnings can be raised and tragedy avoided. Our idea is to try to leverage the extensive network of security cameras present today in most cities.
The third example involves satellites, which are used for long-term flooding incidents, as satellites can pick up radar traces that detect floods. The problem with this is that there is only a limited window every day when the satellite is in the correct position to detect this activity. That’s why we are mainly focusing on using cameras, which enables more rapid short-term warnings to be given. Also, once a flood has happened, you want to evaluate the situation as quickly as possible and so our approach could be helpful for humanitarian causes or for insurance claims etc.”
Abhishek believes that this is the right approach: “Flooding is a global issue that we need to try and solve. OOD generalization is an area that needs much close attention, because the number of trained models is currently quite restricted and limited to what the model has “seen” during training. One could come up with other similar concepts such as an "upside down house" – something that is easy to imagine and even visualize for a person even though no one has ever seen one. However, when the location or shape of the things is changed, AI cannot recognize it. The same is true with flood-related issues – so we need to work towards improving AI model accuracy. I believe that OOD generalization has considerable potential to contribute to many other applications as well.”
Advancing theoretical research
Theoretical research is also a key part of the research focus at FRIPL, with Arkadipta looking at domain adaptation techniques on non-euclidean data for point clouds. This involves training AI models to recognize an object, no matter what variations it is presented with. The objective is to find a cost-effective, faster way of enabling AI training through synthetically generated data compared with the huge overhead of collecting real world data for the same tasks. Abhishek’s theoretical research focus is on physics simulations using AI, initially for manufacturing. By conducting simulations on the shape or structure of vehicles, for example aircraft, it is feasible to reduce the costs of crash testing considerably, combined with improved accuracy and faster production cycles.
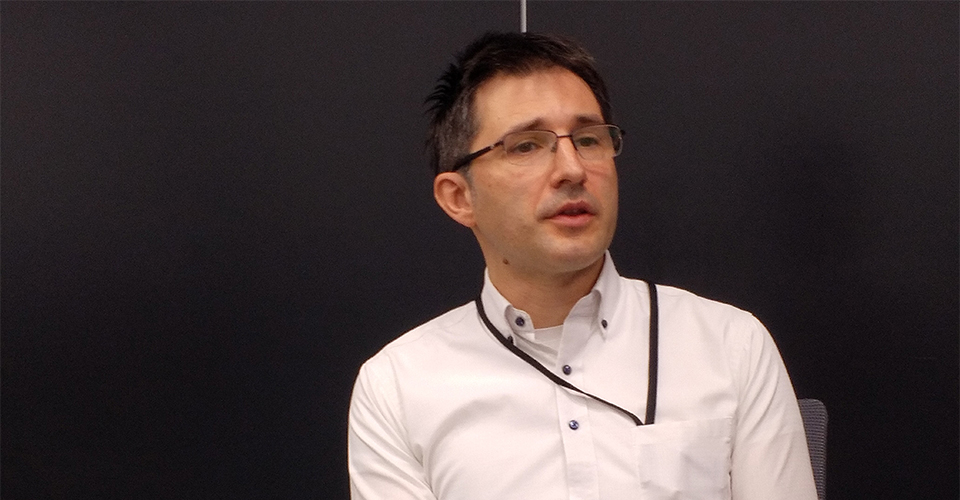
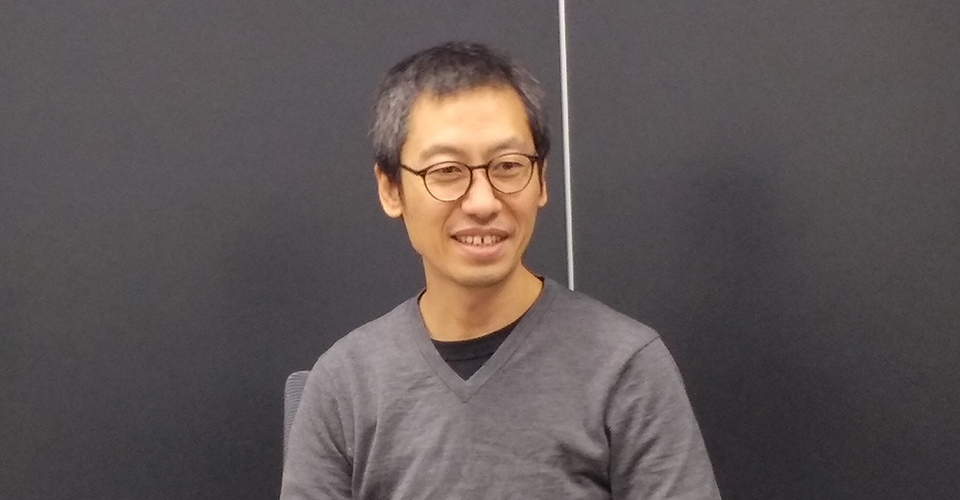
The benefits of international collaboration
The ability to combine experience and knowledge is an essential element of creating both new technology and defining strong use cases, as Serban explains: “Taking the example of the flooding application, India is a country where floods pose a severe social problem, as we have sadly seen too frequently in recent months. Our FRIPL team has a unique perspective on this, which provides us with an essential insight that can help us come up with innovative new approaches.
Despite the miles between us, we have all become accustomed to working together via video conferencing. We have a weekly meeting where everyone reports the progress from last week, what they will do in the next week, possible blockers etc. My role is to facilitate this research by trying to ask the right questions and sometimes to point people in the right direction. Arkadipta and Abhishek are performing the actual research, guided by my questions and also by their experience and curiosity.”
The collaborative approach also extends to starting joint research activities with local Indian universities, which kicked off in April 2022 (*4). The Fujitsu Research and FRIPL teams are working with IIT Hyderabad and IISc to promote innovation in leading-edge AI technologies, as Project Director of the Autonomous Learning Project at Fujitsu’s Artificial Intelligence Laboratory Research Unit, Hiromichi Kobashi, explains: “Our R&D activity with the IIT Hyderabad is focusing on AI technology to discover causal relationships with higher accuracy, while the collaboration with the IISc centers on technology to generate AI automatically through autonomous training in response to various environmental changes.
Our aim is to make a real technological impact for society by enabling sustainable development through these research collaborations. With our AI technology innovation, we want to contribute to a wide range of fields, including drug discovery and new material development. There are also natural disasters like floods, droughts, earthquakes and storms happening worldwide. We hope to solve these important social issues and bring tangible improvements to people’s quality of life though research and development.”
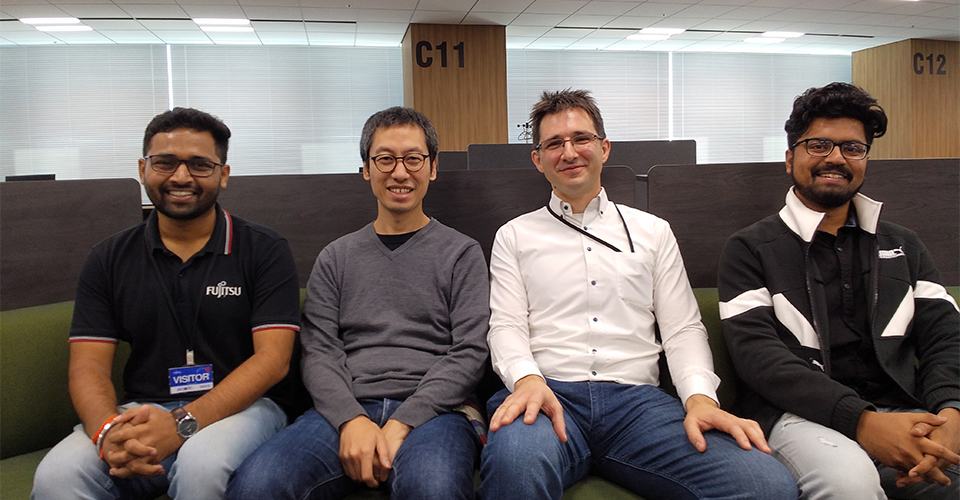
-
(*1)
-
(*2)
-
(*3)
-
(*4)
Fujitsu’s Commitment to the Sustainable Development Goals (SDGs)
The Sustainable Development Goals (SDGs) adopted by the United Nations in 2015 represent a set of common goals to be achieved worldwide by 2030. Fujitsu’s purpose — “to make the world more sustainable by building trust in society through innovation”—is a promise to contribute to the vision of a better future empowered by the SDGs.
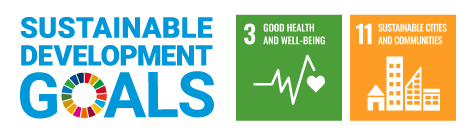