Enabling AI to explain the reason for its judgment
In recent years, along with the rapid development of machine learning technologies such as Deep Learning, various companies have been taking a great interest in artificial intelligence (AI) adoption in their business. However, there is a crucial problem in AI use, which is that “AI cannot explain the reason why it reaches a certain result” to us, humans. This is the so-called black box problem of AI, and it hinders AI utilization in important fields. In order to solve this problem, we have developed our unique and novel machine learning technology, “Wide Learning™.”
Wide Learning™ is a machine learning technology to extract all possible combinations of data items from training data, and to show “important hypotheses that can be key factors for its judgment” in a form that is easy for humans to understand. It is one of the “Explainable AI” technologies. There has been a growing need for Explainable AI since the mid-2010s, and its research has been promoted in the U.S. and many other countries. We can also see the “AI principles” included in the G20 Ministerial Statement on Trade and Digital Economy announced in June, 2019, which was led by the Japanese government. “AI principles” stipulate provisions regarding a “human-centered sense of values and fairness,” “transparency and explainability,” and “accountability” for pursuing “human-centered AI.”
Wide Learning™ is an AI technology that is categorized as a discovery science and takes a different approach from Deep Learning. In this technology, each combination of input data items is called a “hypothesis,” and when a set of input data satisfying a hypothesis is strongly biased to a certain class, the hypothesis is defined as an important hypothesis for classification, which we call a “knowledge chunk.” Wide Learning™ extracts all of the knowledge chunks from an enormous number of hypotheses, and a classification model is built based on them. Researcher Yukiko Yoshida in the Autonomous Machine Learning Project says:
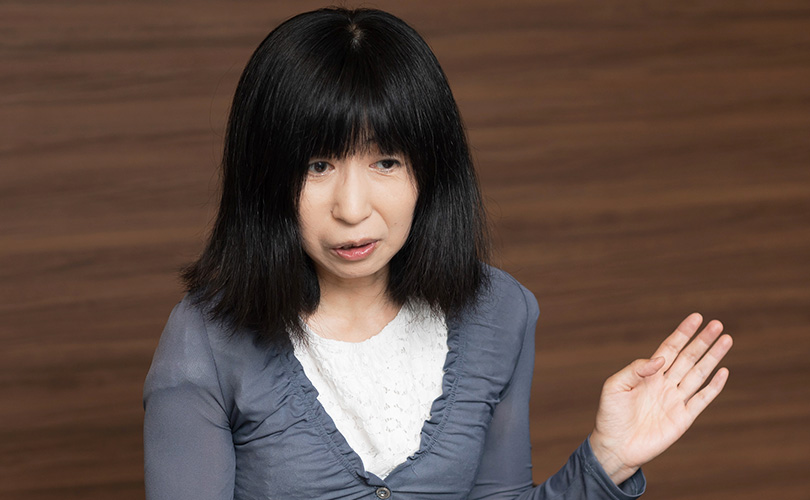
“In a typical AI technique represented by Deep Learning, as its judging process is like a black box, we humans cannot understand ‘why an AI-based system reaches a certain conclusion.’ Consequently, it throws up the question of whether we can adopt the AI judgment result or not. On the other hand, as knowledge chunks, the output of Wide Learning™ are shown in a form that is very understandable for humans, we can understand why the AI reached the conclusion.”
Through knowledge discovery by “enumeration,” hypotheses are created in a human-understandable form.
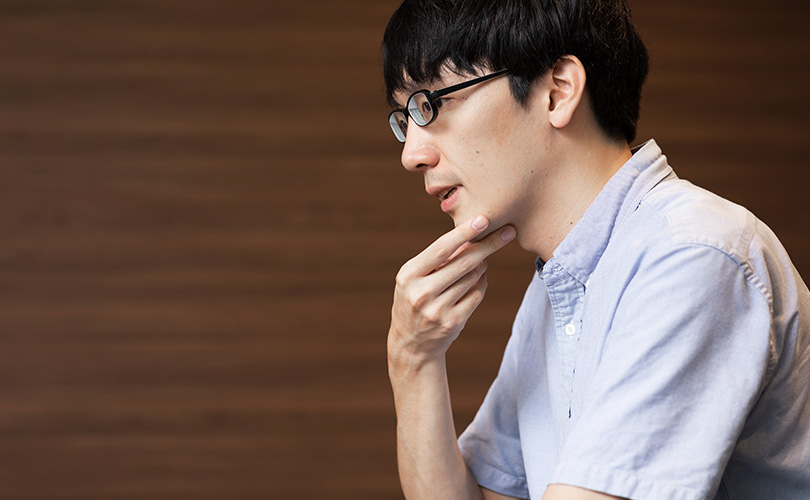
At Fujitsu Laboratories, what kind of methods were used for developing this Wide Learning™ technology? ― Researcher Takuya Takagi explains as follows:
“There are several methods to implement explainability. One of them is to create a locally approximate model, which is easily understandable for humans based on a complex model, like Deep Learning. We took a different approach and tried to create a human-understandable model from the beginning.
Here, we paid attention to the method of ‘enumerating’ a huge number of combinations of data items.”
A “Wide Learning™ system firstly enumerates all possible combinations of data items for finding knowledge chunks to predict the best solution. However, along with the increase in the number of data items, the number of combinations of data items increases exponentially. For many years, researchers have been trying to establish an algorithm that can enumerate at high speed the important hypotheses that are extracted from a huge number of combinations of data items, and they have been producing substantial results. At Fujitsu Laboratories, we tried to develop a technology to eliminate unnecessary hypotheses and enumerate only important hypotheses at high speed, and invented this Wide Learning™ technology. (Takagi)
Our technology is based on the theory of discovery science set up by Dr. Setsuo Arikawa, Professor Emeritus at Kyushu University, in 1990. We enhanced this approach through joint research with Kyushu University. In 2015, Fujitsu Laboratories invited Dr. Arikawa, who is also the former president of Kyushu University, to join and established the Arikawa Discovery Science Center at Fujitsu Laboratories. Based on this center, we tackled the challenge to establish a human-understandable machine learning method, and developed the Wide Learning™ technology. It enabled us to realize a highly transparent AI system based on human-understandable hypotheses, which can work even with just a small amount of data at hand, leading us to discover further knowledge through the enumeration of hypotheses.
Concept of Wide Learning™As shown above, Wide Learning™ enables us to achieve both knowledge discovery and explainability, and we have already been conducting field trials in various business fields. Wide Learning™ especially shows its strength in the business operations where transparency and explainability of an AI system are required such as detection of defects in manufacturing, marketing activities such as advertising, credit examination for financing, and the analysis of disease causes for new drug discovery.
Promoting R&D under the two-team system for “Practical application” and “Technology development”
At present, we are conducting R&D in the Wide Learning™ Research Group, which consists of 13 members in the Autonomous Machine Learning Project of Artificial Intelligence Laboratory.
The Wide Learning™ Research Group is divided into two teams for “Practical application” and “Technology development.” The Practical application team is focused on the commercialization of Wide Learning™ technology, and the Technology development team is responsible for the research and development of basic and cutting-edge technologies of Wide Learning™ for future commercialization. The two teams are collaborating closely.
“The Practical application team communicates with Fujitsu’s sales divisions and SEs for solving customer issues and creating new business with Wide Learning™. When customers are facing difficult problems, researchers visit customers with sales team members to discuss how customers can use Wide Learning™ to obtain the required results. For this purpose, we may directly ask customers about their issues and data at hand. For example, if a customer does not have sufficient data to achieve target accuracy in the learning result, we can combine open data to increase it. In this way, we are preparing various methods to meet customer needs.” (Yoshida)
On the other hand, researcher Yusuke Koyanagi in the technology development team explains the role of his team.
“In the technology development team, each member has their own role. I am taking charge of improving interpretability and persuasiveness of the result derived by Wide Learning™. I am looking for appropriate ways to display and explain the insights obtained through Wide Learning™ in various business fields. ”
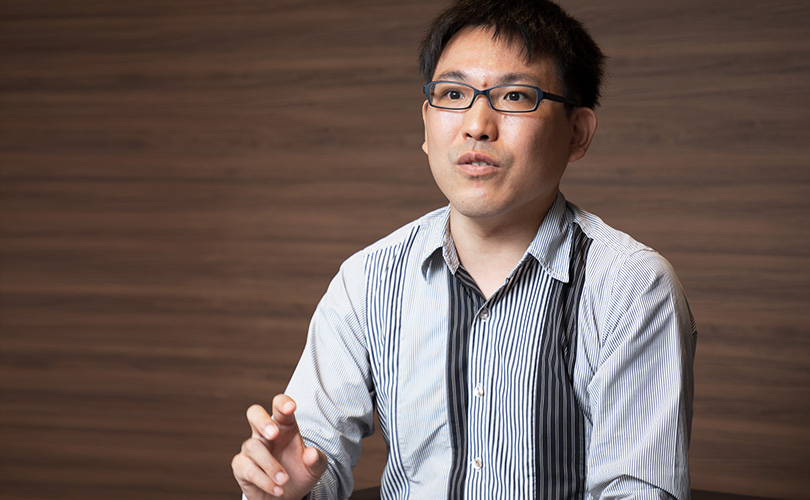
“I also belong to the Technology Development Team and am working on how to accelerate the enumeration of hypotheses, which is a basic technology of Wide Learning™, as well as technological innovation through theoretical and practical approaches, in collaboration with academia. I am also taking charge of the development of tools for utilizing Wide Learning™ in and outside the company.” (Takagi)
And many researchers with diverse knowledge and skills are undertaking further development and the practical use of Wide Learning™, which is a cutting-edge technology in AI/machine learning field.
“We are trying to improve Wide Learning™ and apply it to a wide range of business areas with the knowledge of various research fields, such as natural language processing or knowledge processing. Researchers with a wide variety of backgrounds are communicating and working together as a team in order to proceed to the next step in Wide Learning™” (Koyanagi)
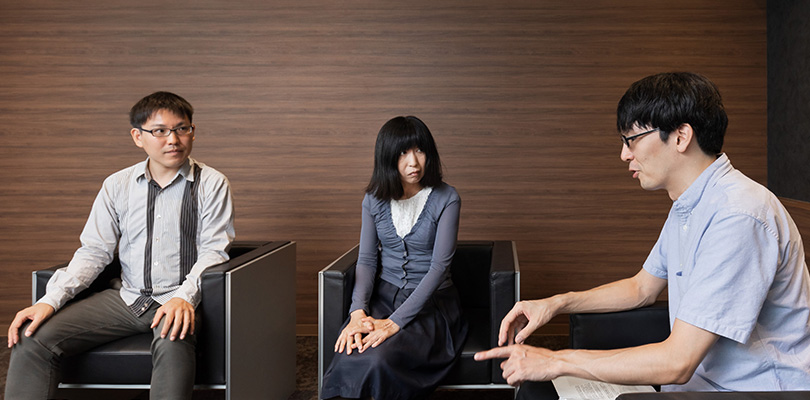
Seeking further development by adding new functions for improving practicability
Since the first announcement in September, 2018, we have executed a lot of field trials. Here, I would like to introduce our future initiatives for meeting further expectations.
“We are trying to expand the applicable fields of Wide Learning™ and obtain substantial results and to accumulate knowledge and know-how for using it to the maximum. During the course of deployment, we may face new issues. We will deal with them appropriately by discussing with Technology Development Team for improving the performance and functions of Wide Learning™. (Yoshida)
In the AI field, clarifying the relationships between data and processing results is also one of the big issues and we are trying to resolve this.
“From now on, we aim to find more precise knowledge and expand its use, by combining the results and hypotheses obtained from various data sets, and explaining it with reference to the meanings of data and background knowledge of applicable fields.” (Koyanagi)
Taking this one step further, we have already installed a new function, which is to propose an appropriate ‘action’ based on the prediction. Wide Learning™, for example, enables us to predict “who has a possibility to purchase the product A, based on a large amount of customer information.” In order to enhance practicability further, the new function, for example, automatically presents and proposes “what action should be taken next for leading a target customer to purchase a target product”, and consequently for increasing the purchase or contract completion rate.
By adding this kind of new function one after another, we will enhance Wide Learning™ technology further. By strengthening the functions for knowledge discovery and explainability through the “enumeration” of all combinations of data items, we will support human decision-making and broaden the AI application area in various business fields.
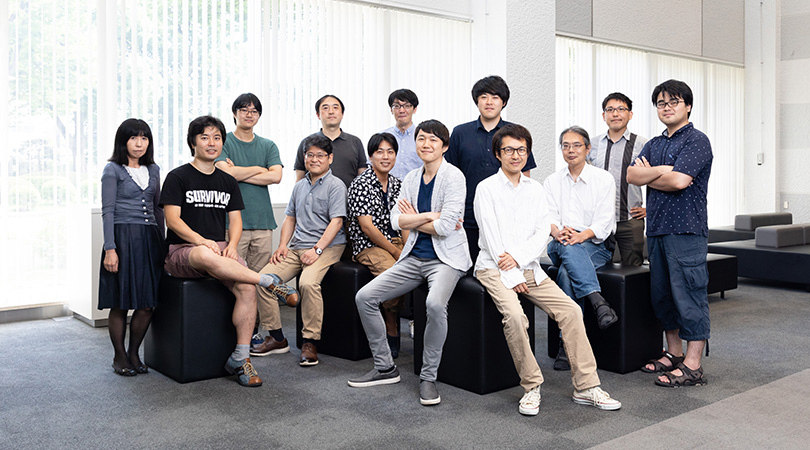