Target! User-friendly yet mathematically grounded AI
Kentaro KanamoriArtificial Intelligence Laboratory
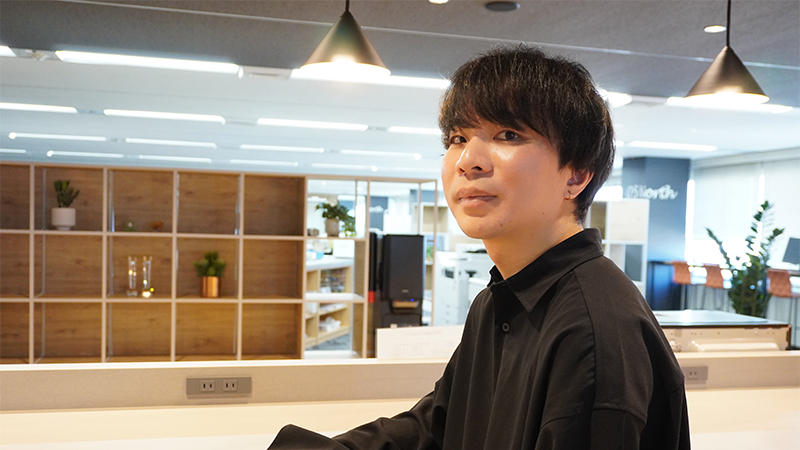
Expressing intelligence and sensibility via mathematical formulas
When I entered university in 2014, the AI boom, which continues to this day, had already begun. I was shocked to learn that various intellectual tasks could be performed by computers. A series of events demonstrating the evolution of AI unfolded, such as deep learning achieving human-level accuracy in image recognition and AI surpassing humans in Go. I majored in information science, which allowed me to study AI-related fields like machine learning and data mining. When I started my research in earnest after joining a laboratory, my supervisor, Professor Hiroki Arimura, introduced me to the concept of "Explainable AI," a technology that can explain the basis of its predictions like a human. I was immediately fascinated.
Thanks to generative AI, AI that can converse in natural language is now commonplace. However, back then, most AI technologies, particularly deep learning, were black boxes. This lack of transparency in how they arrived at their predictions was a major roadblock to practical use. As I progressed in my research, I realized that studying AI is an "attempt to express intelligence and sensibility via mathematical formulas." Explainable AI, in particular, needs to explain the basis of its predictions to human users. Therefore, it is crucial to model concepts related to intelligence and sensibility, such as interpretability and persuasiveness for humans, appropriately as mathematical formulas. This is also where a researcher's skill comes into play. I had always been interested in expressing things and phenomena with mathematical formulas, so I wanted to explore how to express intelligence and sensibility mathematically if such a method existed. Naturally, I then proceeded to the doctoral program in graduate school and conducted R&D on explainable AI.
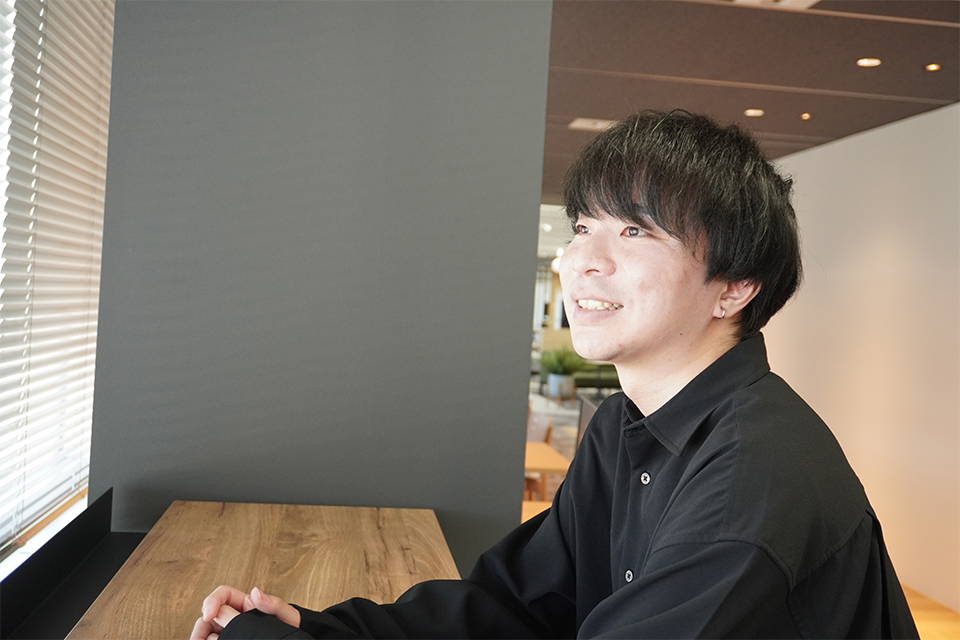
Early success: Internship paper accepted at prestigious international conference
My journey at Fujitsu Research began with an internship. During my second year of master's studies, I started researching explainable AI technology for action explanation (actionable explanation technology), which presents explanations of how to act (take action) to achieve a certain goal. While most explainable AI technologies at the time focused on explaining the rationale behind AI's predictions, actionable explanation technology offered more constructive explanations for humans, such as preventive plans to avoid the onset of diseases. I felt this technology held great potential for practical application.
After presenting my initial research findings at a research workshop, I learned that Fujitsu Research was also interested in the same research theme. I applied for an internship at Fujitsu and focused on researching actionable explanation technology. The paper summarizing the results of my two-month internship was accepted at AAAI, a top-tier international conference in the field of AI. It also received high acclaim both domestically and internationally, including the Japanese Society for Artificial Intelligence (JSAI) Best Paper Award, which significantly boosted my confidence as an aspiring researcher.
In additional, my internship experience at Fujitsu Research allowed me to connect with supportive senior researchers within the company and collaborate with Ken Kobayashi (currently Associate Professor at Institute of Science Tokyo) and Yuichi Ike (currently Associate Professor at Kyushu University) – both connections which have become a valuable asset in my subsequent research career. Our collaboration continues to this day, and this year, our paper on actionable explanation technology was accepted at ICML, another prestigious international conference in the field of machine learning.
Causal Decision-Making Assistant: A technology born from real-world challenges
After completing my doctoral program and joining Fujitsu, I became involved in the R&D of decision-making technology, using explainable AI, and supporting its practical application. Shortly after joining, I learned that a business division within the company was interested in actionable explanation technology, believing it held business opportunities. Examples included improving yield rates in manufacturing plants and suggesting measures to improve employee work-life balance and productivity. They agreed to use the technology I had developed and conduct performance evaluation experiments for practical application.
However, repeated performance evaluations revealed various challenges in putting the technology to practical use. The biggest hurdle was processing time. In the academic research world, one can assert superiority based on experimental results obtained with stable computing resources and manageable benchmark data. But in real-world applications, computing resources are limited, and the target data may be large-scale or incomplete, preventing the developed technology from fully demonstrating its capabilities. While it was frustrating to discover that the technology I had developed wasn't practical in real-world settings, it was also a valuable experience as a corporate researcher to identify challenges that wouldn't have been apparent solely from writing papers at my desk.
I repeatedly discussed the issues identified in the performance evaluation experiments with my superiors and colleagues, striving to apply the lessons learned to the next project. In March 2024, we were able to publish a technology called "Causal Decision-Making Assistant" on the Fujitsu Research Portal (*1), which combines the existing actionable explanation technology with statistical causal discovery technology. This technology analyzes causal relationships between elements in the data to recommend optimized measures that are most effective in achieving goals while avoiding negative side-effects on other elements. Since its publication, I've increasingly heard from the business division that the technology has been very well-received by clients. One of my motivations for joining Fujitsu was to create technology that could contribute to society. I feel a great sense of accomplishment knowing that the technology I developed is generating interest and being used in practice.
Relaxing with photography and reading
I've enjoyed photography as a hobby since my student days. I still use the single-lens reflex camera I bought with my part-time job earnings in university. I love taking pictures of people and animals. Animals, in particular, are challenging because their movements are unpredictable. It's rewarding when I can capture a satisfying photo after much experimentation and effort. I find that the process of repeated trial and error in pursuit of a satisfying result is similar to research activities.
On my days off, I like to relax and work on personal projects at a cafe. Recently, I've been reading books on humanities topics such as cognitive science and philosophy. Working on explainable AI has sparked my interest in exploring what constitutes a truly understandable explanation for humans, leading me to study related fields.
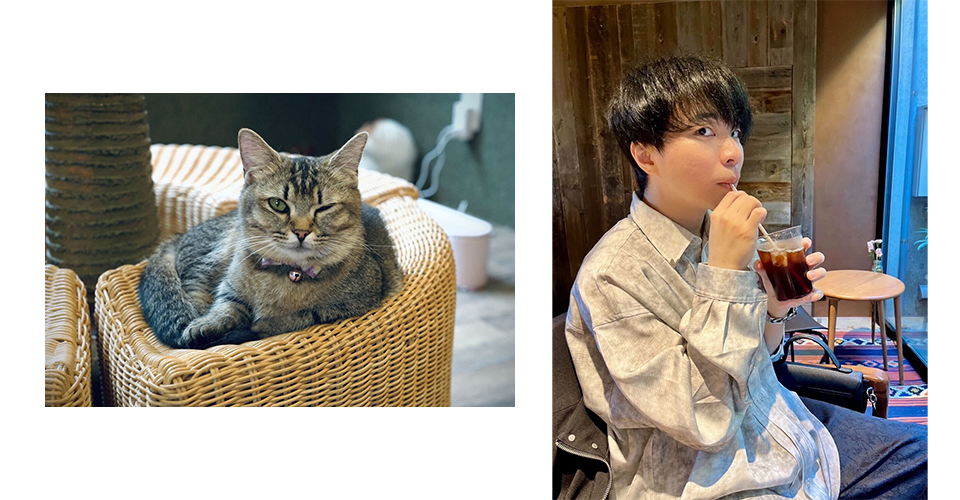
(Right) My favorite cafe.
Striving to balance academia and corporate research
In addition to my R&D work as a corporate researcher, I'm also engaged in research activities as a first-generation member of the ACT-X project, "Innovations in Mathematical and Information Sciences to Build the Next-Generation AI." This is a competitive research program for young researchers promoted by the Japan Science and Technology Agency (JST), aimed at discovering and nurturing outstanding young researchers to overcome important challenges facing Japan. With the support of my company and superiors, I applied and was accepted. Within this program, I'm tackling research topics related to actionable explanation technology. My goal is to become a well-rounded researcher, establishing a presence within the academic research community, primarily centered around universities, while also producing research results with a tangible impact on society.
With the rise of generative AI technologies, exemplified by large language models, I believe the ideal form of explainable AI and the direction of research are also transforming. While generative AI services like ChatGPT are becoming widely accessible, issues such as hallucinations pose reliability challenges. I feel there are still hurdles to overcome before generative AI technologies can be utilized for critical decision-making in real-world scenarios. Rather than rejecting new technologies or discarding older ones, I aim to realize AI technology that truly supports humans by combining the user-friendliness of conversational generative AI with the rigor of mathematically-grounded explainable AI, effectively leveraging the strengths of both.
Messages from colleagues
Kentaro possesses top-tier research abilities within the Artificial Intelligence Laboratory, evidenced by achievements such as his ICML 2024 spotlight paper and the JSAI Best Paper Award. He has a broad perspective that extends beyond his area of expertise and the ability to solve problems with diverse ideas. I am very excited to see the contributions he will make at Fujitsu in the years to come. (Takuya Takagi, Senior Research Manager, Artificial Intelligence Laboratory)
-
(*1)Fujitsu Research Portal lets you try out Fujitsu's advanced AI technologies. If you are interested in Causal Decision-Making Assistant, please contact us here.
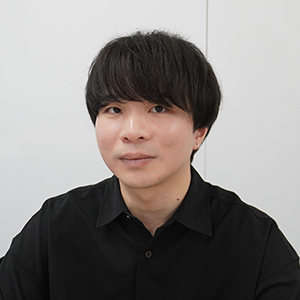
Titles, numerical values, and proper nouns in this document are those reported when this interview was made.