With the recent global trend toward the full-scale use of AI systems, many companies are rushing to raise work efficiency and productivity by introducing AI systems. However, we see a new problem occurring, which is the gradual deterioration of AI model accuracy during target system operations. Why does the accuracy deteriorate? Katsuhito Nakazawa, Research Manager of the Trusted AI Project in our Artificial Intelligence Laboratory says:
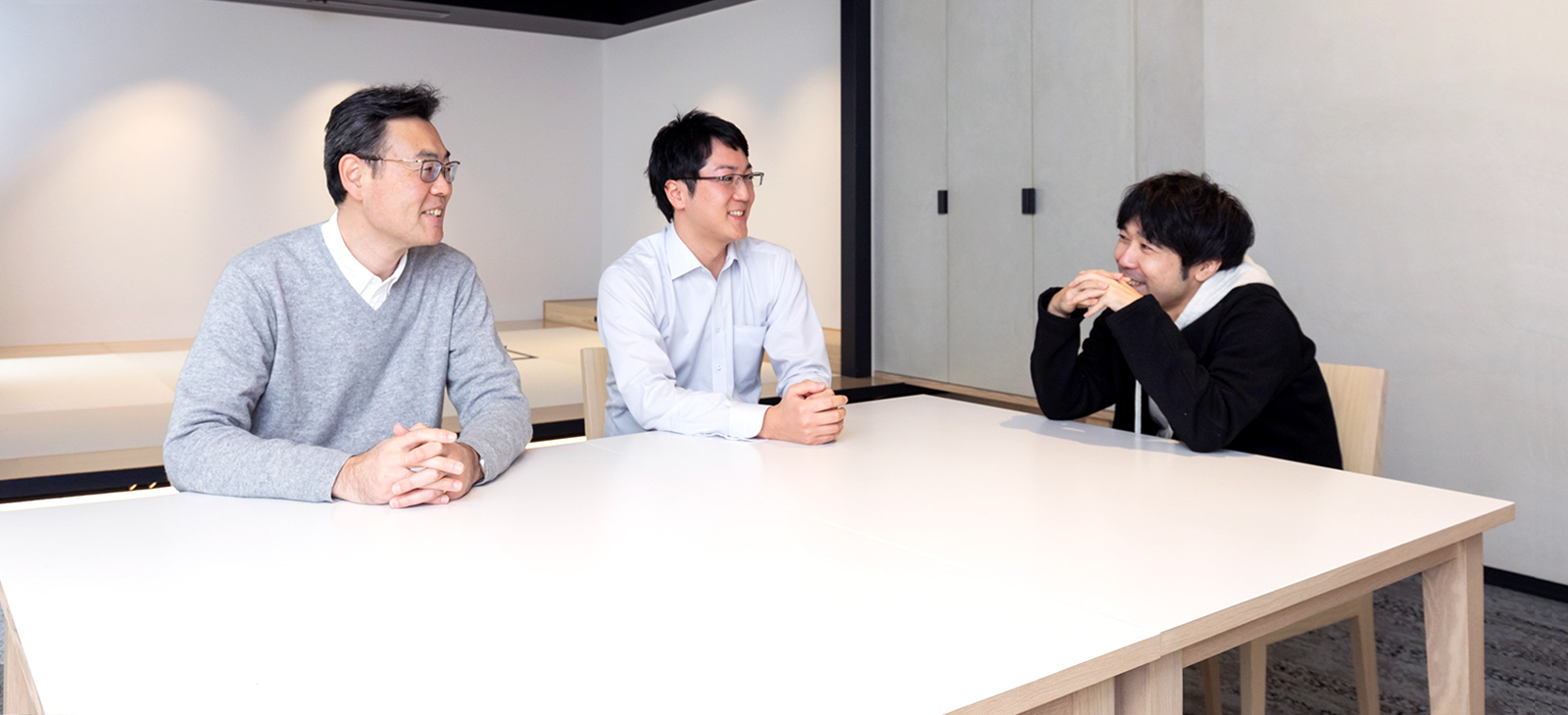
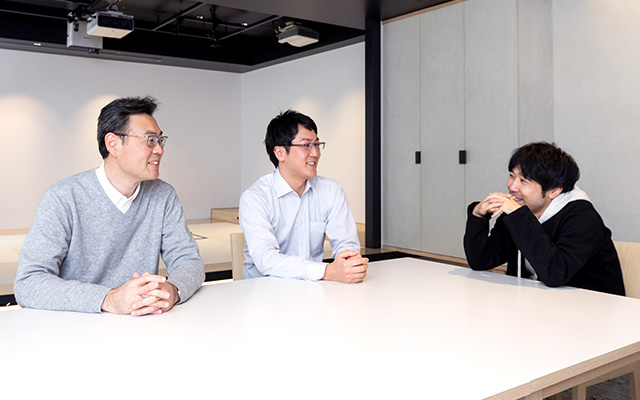
While the number of companies using Artificial Intelligence (AI) for their business is increasing, a new problem has appeared, which is the “deterioration of AI model accuracy during long-term system operation.” In order to solve this issue, Fujitsu Laboratories’ Artificial Intelligence Laboratory developed a ground-breaking technology to detect the deterioration of AI model accuracy and restore it automatically. We interviewed the project members, who invented this world-first technology called “High Durability Learning,” about its development process and outstanding features.
Posted on February 13, 2020
MEMBERS
Katsuhito Nakazawa
Research Manager
Trusted AI Project
Artificial Intelligence LaboratoryYasuto Yokota
Trusted AI Project
Artificial Intelligence LaboratoryYuhei Umeda
Research Manager
Autonomous Machine Learning Project
Artificial Intelligence Laboratory
AI system operation issue involving AI model accuracy deterioration
“When we develop an AI system, we create an AI model, which is used for a target business system, by preparing the training data in that field and applying repeated machine learning. Then, we input the target business data into this AI model to make it output the processing results such as predictions or decisions on given cases. However, over the course of long-term operation, its external environment changes and the content of new data input into the AI model keeps changing from its initial training data. As a result, the AI model becomes outdated and its accuracy deteriorates.”
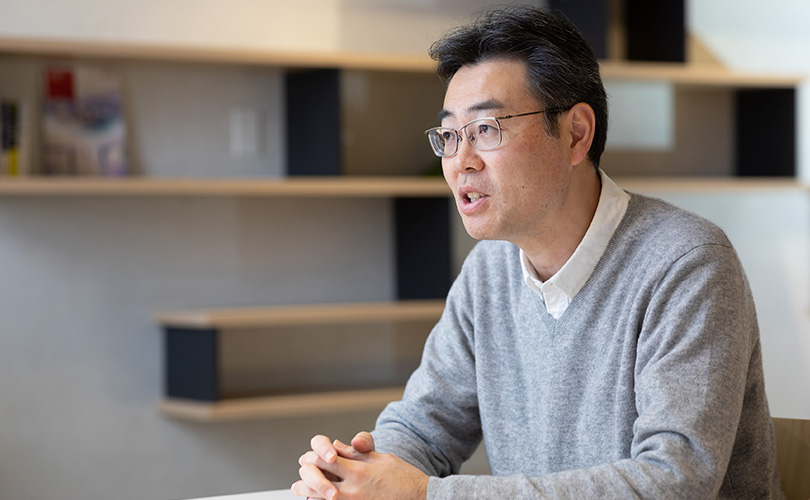
These kinds of problems may arise in various fields where AI systems are expected to be introduced and used. For example, financial institutions are examining AI adoption for corporate credit risk assessment. As AI evaluates the loan risk based on financial statements, etc., due to fluctuations in exchange rates and interest rates, the AI model’s prediction accuracy lowers.
In order to solve these issues, we have to maintain the AI model regularly. However, this is not so easy.
“In order to keep AI accuracy, we need to perform retraining by using training data with annotations. However, this involves a lot of cost and time to annotate manually for retraining. On the other hand, if we keep using it without doing maintenance for a long time, AI accuracy goes down and it becomes impossible for AI to make a correct prediction, which may cause serious damage.” (Nakazawa)
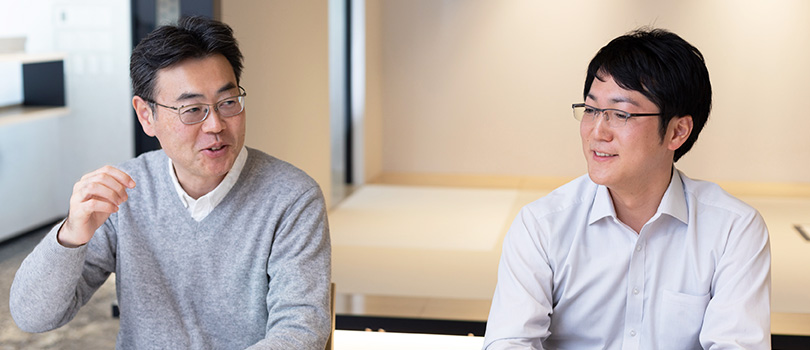
New technology to automate detection of deterioration and restoration of AI accuracy
These issues of AI system operation have already been discussed in several industries and they are insisting on the need for concrete prevention measures. Against this background, Fujitsu Laboratories began to develop a technology automatically to recover the AI accuracy when it deteriorates. As a result, we announced the world’s first technology named “High Durability Learning” in October, 2019.
With a conventional AI operation method, unless correct labels are put to the input data in system operation, it becomes difficult to judge whether the AI accuracy is lowering or not. Therefore, experts had to add the correct labels to newly input data regularly and confirm the accuracy. If they identified a deterioration, they retrained the model. This work involved huge costs. On the contrary, “High Durability Learning” uses a mathematical space called a “DT space (Durable Topology Space)” where the data distribution characteristics are shown as masses of data, and while monitoring the changes of the shapes of these masses with time, it determines the correct answer (annotation) for each piece of input data. Based on these annotation results, the annotation is performed again in the original data space. Then, comparing the difference from the initial classification, it can automatically estimate the deterioration rate of an AI model.
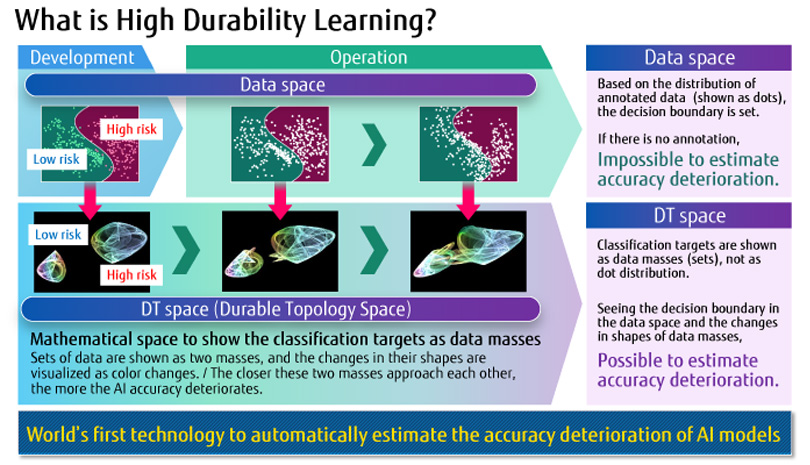
In this way, “High Durability Learning” enables the accuracy deterioration to be detected and the accuracy automatically to be recovered without performing annotation by experts and retraining. According to our verification results using an actual AI model, the rate of detection error was only 3%. And when we used a conventional method, the prediction accuracy of the AI model lowered to 69% from its initial rate of 91% after one year. On the contrary, when we apply “High Durability Learning” technology, the AI model’s prediction accuracy can be kept at 89%. As the automatic restoration function can decrease the retraining frequency by 90% and retraining costs by 80% or more, it becomes possible to cut the total retraining costs to one hundredth or less.” (Nakazawa)
Another highly significant feature of “High Durability Learning” that we emphasized in its development is “versatility.” As the High Durability Learning system is not environment-dependent, it can be used for various AI systems as an add-on function.
“As various AI models are used in business, we considered that it is important to develop a function, which, can be applied to any kind of AI model without limiting the type of input data and which can be used directly as an add-on with versatility. We have already finished the verification proecess by using representative AI models and algorithms such as a deep neural network (DNN), a support vector machine (SVM) and Random Forest, and we are planning to expand its application range further.” (Nakazawa)
Project started with Ideathon (brainstorming) for issue-solving technologies
Now, “High Durability Learning” is attracting attention as a technology to solve issues in AI system operation. This R&D project was launched by an internal working group, which was set up in the autumn of 2018. Based on the research results of this working group, the research group was formed and has been led by Research Manager Nakazawa, who had been engaged in AI quality management and Explainable AI-related technologies such as Knowledge Graph.
“Fujitsu is not only an IT company but also a manufacturing company, for which high levels of technological strictness are required. Therefore, I considered that it is our role to present a firm solution for the AI accuracy deterioration problem. We started with a brainstorming session in which researchers from the Trusted AI Project participated to share ideas, coming up with about 20 initial ideas. After discussing their feasibility for about two months, we started the R&D project in full swing.” (Nakazawa)
Through these activities, we came up with the “DT space” technology. And, it was Yuhei Umeda, Research Manager of Autonomous Machine Learning Project, who moulded this idea into shape. The feature of this technology is to grasp the data changes more mathematically and topologically.
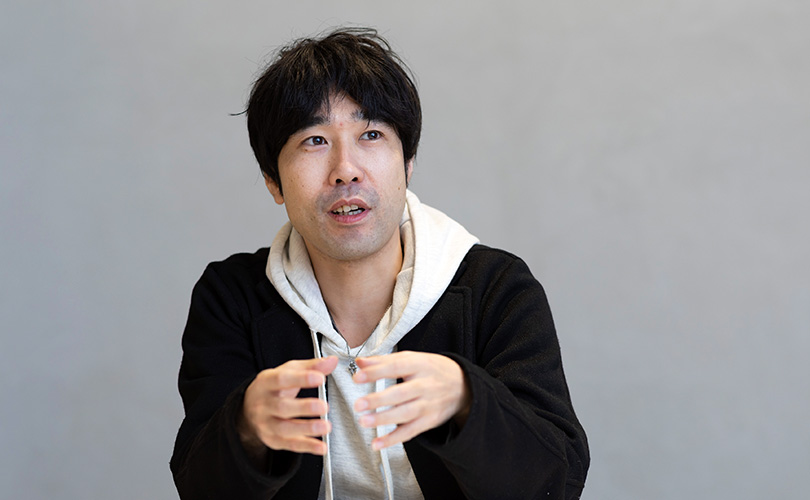
“In a data space of general machine learning, we can only trace the movement of each piece of data one by one. However, in order to grasp the characteristics of the whole data, we need to grasp a set of data as a mass, and to trace time-series changes in its shape mathematically with a bird’s-eye view. We paid particular attention to the information on the shapes of two masses of classified data and tried to analyze them by using “Topological data analysis (TDA),” and continued the development of a machine learning technology. Then we reached the concept of a “DT space.” (Umeda)
It was Yasuto Yokota, one of the initial members of this project, who took charge of designing and implementing an algorithm for identifying the data characteristics with a high degree of accuracy, by using this DT space. He has experience in the development of applied technology of Deep Learning and made efforts to resolve relevant issues in cooperation with Fujitsu’s business unit. Making the most of his implementation ability, he created its program.
“We tried to design the algorithm for extracting important parts from the topological mass of data. It was really tough for us to create a prototype without a design document and to improve it through trial and error.” (Yokota)
World’s first technology developed by researchers with diverse talents
As a result, we were able finally to announce “High Durability Learning” as a world-first technology. We feel really grateful for our company’s environment that allows us to promote R&D on basic technologies in collaboration with Fujitsu’s business units, who have an excellent grasp of customer needs.
Yokota continues as follows.
“Many companies and academic institutions are working on the research and development of AI. And, most of them focus on the development of machine learning algorithms and AI models. In this situation, as Fujitsu Laboratories is a member of the Fujitsu Group with its many business divisions, we can get the information on problems that our customers are facing in their AI system operation, and take an approach from an “operational” perspective.
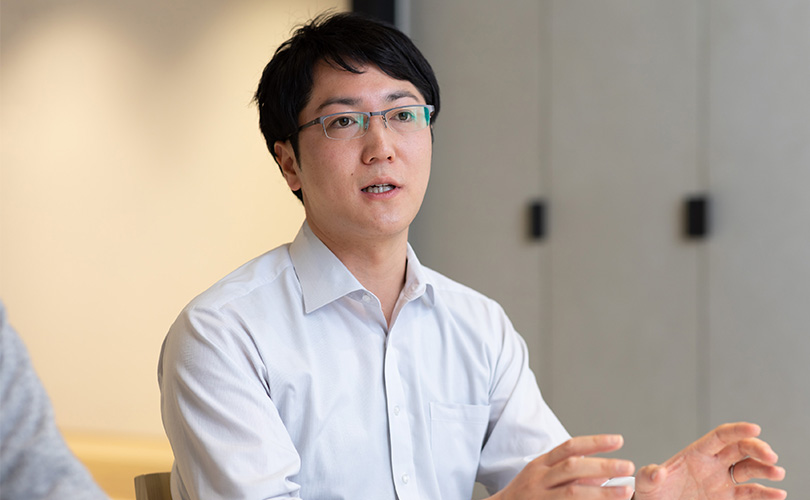
Fujitsu Laboratories has many researchers with an abundant knowledge about the situations of on-site work, as well as mathematics, algorithms and designing for system implementation. They also have diverse professional backgrounds. Not only researchers with an in-depth experience in leading-edge technologies but also ex-engineers recruited through Fujitsu’s business units, or those employed as mid-career recruits like Yokota, who previously worked outside the Fujitsu Group. The “High Durability Learning” technology was the fruit of the collective efforts made by this wide variety of talent and expertise.
We are now advancing the verification process for applying “High Durability Learning” to AI systems in various fields such as credit risk assessment in the financial industry, merchandise image classification in the retail industry, and character recognition of delivery slips in the distribution and logistics industry. We are also planning to enhance other functions such as a dashboard to visualize the result of AI accuracy monitoring.
“If we can avoid AI accuracy deterioration and assure stable system operation, companies will introduce and use AI systems more actively. We are convinced that our mission is to realize trustworthy AI systems that can adapt to the changes in socio-economic situations and make correct decisions with fairness and explainability.” (Nakazawa)
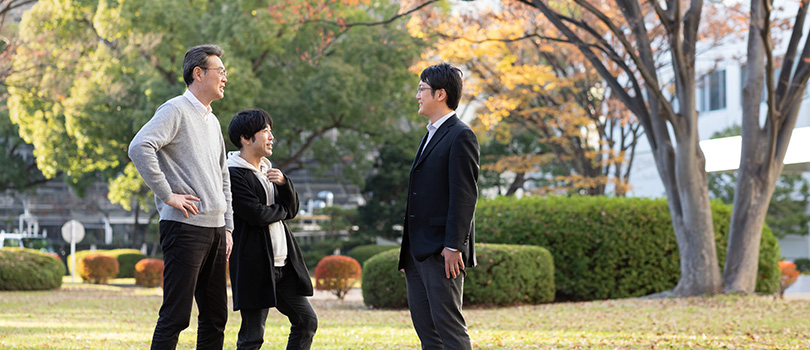