Predictive maintenance
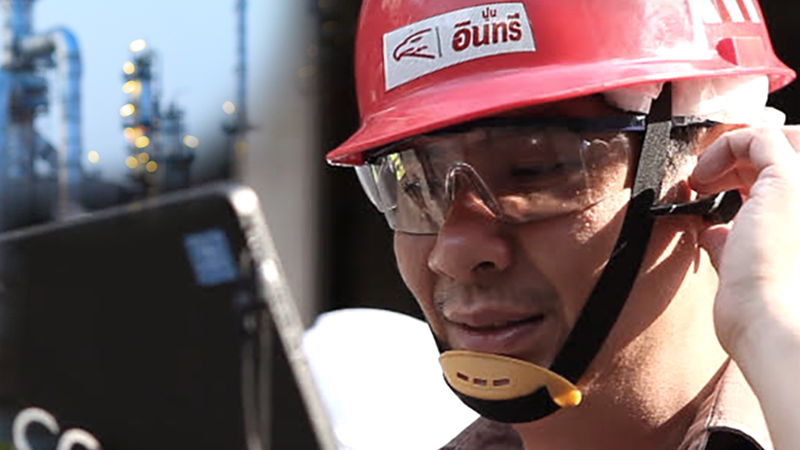
Preventing Equipment Breakdown with Machine Learning
When there is a critical breakdown of production equipment in a factory, the consequences can be costly.
An unscheduled major repair on a machine could shut down an entire production line and, on average, it would take two days to get the malfunctioning equipment back into service.
Predictive analysis
Predictive analysis with machine learning can reduce expensive downtime and maintenance while improving the overall equipment effectiveness (OEE) of the machines. That’s why it forms a key part of the technologies that make up our Smart Factory digital solutions.
Predictive analysis with machine learning is an application of AI, which can alert plant managers and engineers to anomalies in the functioning of machines. This, in turn, could foretell problems ahead, including a critical breakdown of the equipment.
Predictive analysis also can help the machines run more smoothly by optimizing the replacement schedule of parts and of the machines themselves. At the same time, it can prolong the life of parts and reduce excess spare parts inventories that the plant needs to keep on hand.
But the success of predictive analysis and machine learning doesn’t happen overnight. It requires time to yield results.
Collection of data
“For the entire smart factory, the first step is about collection of data,” says Alfee Lee, DX Officer (DXO) ASEAN+I (India). “In order to achieve predictive analysis, the first step is to capture the behavior of the machines, and to integrate different machines’ data points to develop a pattern for analysis.”
Sensors are deployed on all machines to collect data, forming a broad IoT infrastructure in the plant. This data is then sent via Wi-Fi to company headquarters for analysis. Plant managers and senior engineers can also see the data updated in real-time at a remote operation center, which forms the hub of the Smart Factory. And the inspectors, doing regular digitalized walk-by inspections of machines, also feed data into the system, which is centrally analyzed and monitored.
The more data the system processes, using machine-learning algorithms, the more it can predict potential problems and maintenance issues with the plant’s equipment.
Siam City Cement Co.
Machine learning and predictive analysis are part of the Smart Factory Fujitsu rolled out for the Siam City Cement Co., or SCCC, which launched in late 2017. Fujitsu worked with a partner on the predictive analysis piece.
Early results from the implementation promise a 2% improvement in annual OEE and a decrease in maintenance costs by 10%, said the plant operations VP. In one estimate, Fujitsu said the preventative maintenance, aided by machine learning and other Smart Factory technologies, could save the plant 240 million Thai baht (US$7.7 million) per year by avoiding machine breakdowns.
But while machine learning reduces unscheduled downtime and maintenance costs, this is only one of the tools that plant managers and engineers have at their disposal to help them make better decisions. Human judgment and management will always be part of the mix in Smart Factories like SCCC.
“When we have machine learning, the human being is still the decision-maker,” says Fujitsu’s Lee.