Fujitsu Research of America
AI Lab
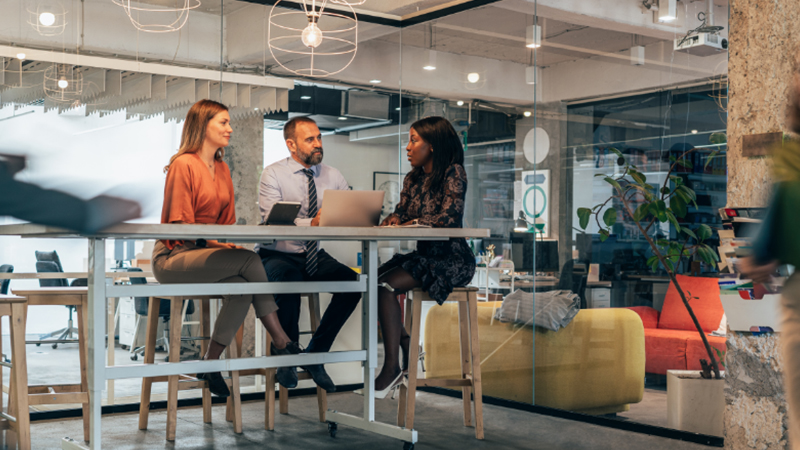
Collaborating with people to create new value
Our focus is on realizing a sustainable society by developing cutting-edge AI technologies that not only create new value but also contribute to the transformation of society and business.
Automatic machine learning, commonly known as 'AutoML,' holds great promise in democratizing the use of machine learning (ML) by automating a significant portion of the tasks typically performed by data scientists. However, the vast search space of potential pipelines presents a challenge, often leading to the generation of suboptimal or no pipelines, especially when dealing with large and complex datasets.
Fujitsu Research has actively tackled this issue by developing a unique AutoML technology called 'Fujitsu AutoML.' Leveraging a collection of pre-existing datasets and their human-created pipelines, this technology enables the efficient generation of high-quality pipelines for new datasets with predictive tasks. With Fujitsu AutoML, data scientists can rapidly create and modify AI models, as the code is provided along with detailed explanations. Moreover, citizen data scientists can easily create the desired AI models as well.
Researchers in the AI Lab
Avraham Cooper (Avi)
Multi-model foundation models,
computer vision,
large scale AI system designHiromichi Kobashi
Machine Learning (ML),
distributed systems,
databasesIan Mason
Machine Learning (ML): domain adaptation, continual learning,
foundation modelsJin Yamanaka
Machine Learning (ML), Computer Vision
Kanji Uchino
NLP (information retreival,
semantic web),open education,graph AI,
AI ethics,AutoMLLei Liu
Natural Language Processing,
(NLP),Machine Learning (ML),
and communication networksMaria Xenochristou
Machine Learning (ML),
Computer Vision,
multimodal learningMichael McThrow
Machine Learning (ML), AutoML, graph AI,
databases,programming languages
Mehdi Bahrami
Natural Language Processing (NLP), Machine Learning (ML)Ramya Srinivasan
Machine Learning (ML),
computer vision,AI ethics,
Natural Language Processing (NLP),AI and creativityShailaja Sampat
Natural Language Processing (NLP),Computer Vision,
multimodal learningSo Hasegawa
Machine Learning (ML),
Computer Vision,
generative models
Trishala Ahalpara
Machine Learning (ML),
customer segmentation, AutoML,bias in AI,
feature selectionWei-Peng Chen
Machine Learning (ML),
optimization,
mobile networksWing Yee Au
Machine Learning (ML),
relational and graph dataXavier Boix
The Self-Improving Machine Learning group
Director of Research
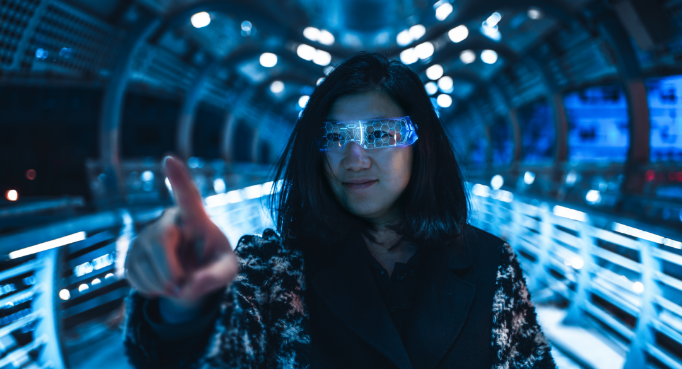
Publications
- Juhan Bae, Michael R Zhang, Michael Ruan, Eric Wang, So Hasegawa, Jimmy Ba, Roger Grosse, “Multi-Rate VAE: Train Once, Get the Full Rate-Distortion Curve”, International Conference on Learning Representations (ICLR) 2023
- So Hasegawa, Masayuki Hiromoto, Akira Nakagawa, Yuhei Umeda, “Improving Predicate Representation in Scene Graph Generation by Self-Supervised Learning”, Proceedings of the IEEE/CVF Winter Conference on Applications of Computer Vision, 2023
- Ramya Malur Srinivasan, Hiroya Inakoshi, Kanji Uchino, “Leveraging Cognitive Science for Testing Large Language Models”, 2023 IEEE International Conference On Artificial Intelligence Testing (AITest), 2023
- Youzhi Luo, Michael McThrow, Wing Yee Au, Tao Komikado, Kanji Uchino, Koji Maruhashi, Shuiwang Ji, “Automated Data Augmentations for Graph Classification”, ICLR2023, 2023