- Table of Contents
-
- For LLM to achieve learning capabilities equivalent to humans, it requires the computational power of Fugaku
- The processing capacity of Fugaku that has not yet been fully utilized will be pulled up to 5 times
- As AI capabilities approach those of humans, transparency and accountability become more important
- Enjoy new AI society with expectations
For LLM to achieve learning capabilities equivalent to humans, it requires the computational power of Fugaku
—ChatGPT and other generative AIs are drawing a lot of attention. AI has unlimited potential, possibly exceeding human capabilities, and its impact is considerable. Against this backdrop, Fujitsu also announced an initiative to utilize the supercomputer Fugaku for LLM development.
Kobayashi: In simple terms, generative AI is AI that can generate content such as text and images in the same way as humans. Typical examples are AI that generate content such as sentences and images, but there is also AI that can generate music and videos. AI that can generate natural sentences like ChatGPT is called LLM.
Fujitsu has been engaged in LLM research and development for some time, but not on a large scale. However, the emergence of ChatGPT had a huge impact on the world, and there was a sense of crisis that Japan might fall behind in research and development in this field. In addition, the language used in LLM development is mainly English. If we did not work on the development of LLM in Japanese-language now, it was anticipated that the accuracy of LLM in Japanese would remain low in the future.
Therefore, instead of continuing our research on the scale of the past, we have started to work on the research and development of LLM on a larger scale. Specifically, Tokyo Institute of Technology, Tohoku University, RIKEN, Nagoya University, CyberAgent Inc. and Fujitsu are collaborating to create a new Japanese LLM on an unprecedented scale using Fugaku.
—Because of this background, some people believe that industry and academia, including Fujitsu, have cooperated in this effort to develop Japanese ChatGPT using Fugaku. Is that a correct perception?
Kobayashi: This may sound surprising, but the goal of this initiative is not to develop LLM like ChatGPT; the goal is to create an environment that will enable the development of LLM. By the time this project comes to an end, we will have gained a variety of technical insights for developing LLM. Fugaku will be used to build that environment. To achieve human-like learning with LLM, large-scale deep learning based on huge amounts of data is required. High-performance computing resources are essential for this, and Fugaku’s processing power will be utilized for this purpose.
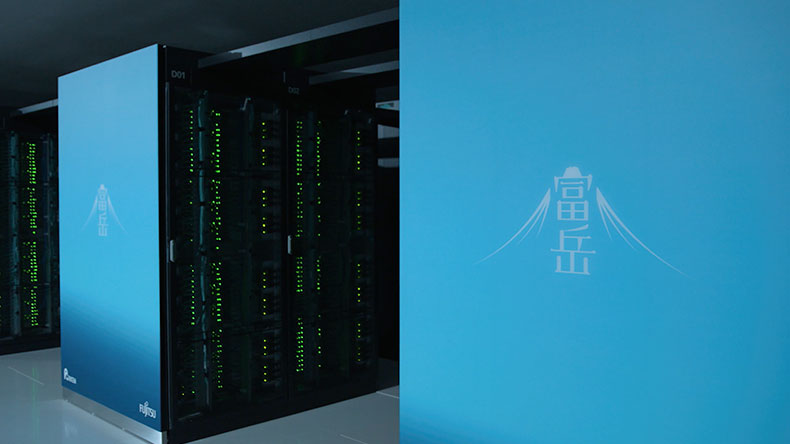
The processing capacity of Fugaku that has not yet been fully utilized will be pulled up to 5 times
—You mentioned that a huge amount of data needs to be learned, but what is the exact data amount?
Kobayashi: A vast amount of data, that is for sure. However, there are still many things to consider, so I cannot give you a specific amount at this time. However, we do know the minimum amount of data required for the amount of computation during training. It has been confirmed that emergence is demonstrated when a huge amount of computation (1023 FLOPs) is used for learning in a base model such as LLM. Then, the LLM will suddenly be able to do things that it could not do at all before, even without being taught. In other words, in order to make LLM as good as humans, it is necessary to make them learn 1023 FLOPs, which is an amount of computation that is impossible for a normal computer (6,000 years of computation on a single smartphone).
We know that if we let AI learn that amount of data, LLM’s performance will improve dramatically, and it will acquire language abilities it did not have before. Moreover, it is believed that AI will naturally acquire various abilities as the amount of data and computation increases.
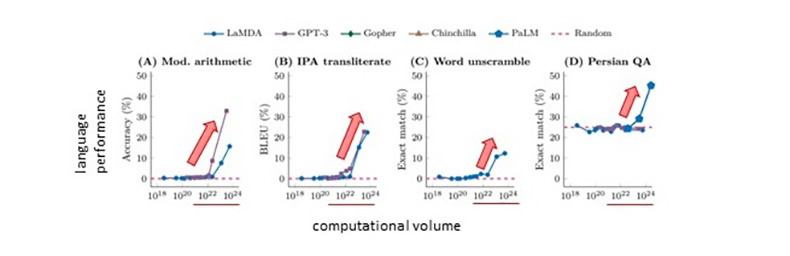
This is why we are utilizing Fugaku. Fugaku is designed to be used as a supercomputer for various purposes. However, LLM learning was not envisioned as an application. Before this project began, Fugaku was using only 10% of its capabilities for LLM learning. Therefore, we are optimizing the learning software for LLM used in Fugaku to increase its capability to 50%.
—How do you raise 10% to 50%? This is a five-fold increase, isn’t it?
Kobayashi: Parallel distributed processing technology is the key. When the LLM is this huge, the learning data is also huge. The LLM is also so large that it cannot be stored on a single server, but must be divided and stored on many servers. In this case, tens of thousands of servers will be used. During learning, these servers need to run in parallel while communicating closely with each other. This is a complex process involving many factors. For example, how to partition and deploy the training data and LLM, determine the order in which to execute the vastly divided training process, and communicate and share the intermediate results computed by the many servers during the training process. Parallel distributed processing is a technology that optimizes and efficiently handles these complex processes. By developing the technology and software for the LLM study, we aim to increase Fugaku’s capacity by 50%.
Fugaku has approximately 160,000 nodes (one node is equivalent to one server), and this initiative will use one-fifth of that figure, approximately 30,000 nodes. It takes about 150 days to process the huge amount of calculations (3×1023 FLOPs) required to achieve LLM equivalent to GPT-3 on 30,000 nodes. One of our goals is to shorten this time to about 30 days by developing and optimizing a parallel distributed processing technology for LLM.
Another goal is to improve the language performance of LLM, particularly with respect to the Japanese language. In order to improve the Japanese performance of the LLM, we need to prepare a huge amount of Japanese data. Not just any data can be used, but good quality data must be collected. In addition, based on the characteristics of Japanese as a language, pre-process the data so that LLM can easily understand Japanese. It is also important to adjust the LLM so that the output sentences are appropriate and harmless to humans. Because of the various factors that are expected to influence this process, it is difficult to train the best-performing LLM from the outset. We plan to start with a small-sized LLM, about one-tenth of the final LLM, and gradually improve its performance through repeated experiments.
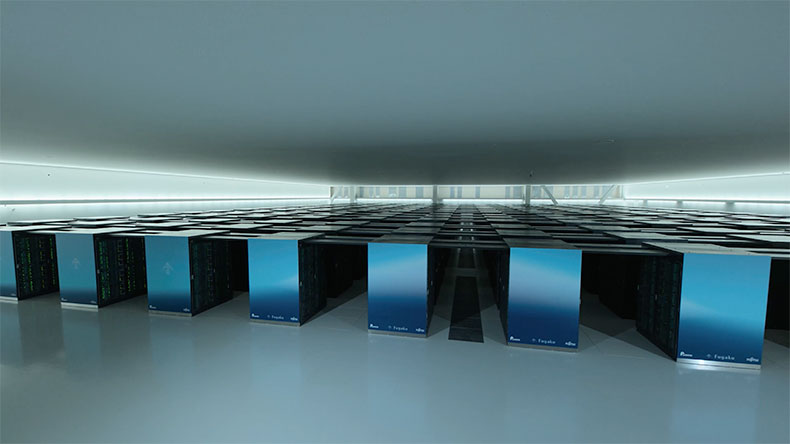
As AI capabilities approach those of humans, transparency and accountability become more important
—I heard that Fujitsu’s Artificial Intelligence Laboratory is working on research and development of various LLM, including LLM in areas that ChatGPT cannot cover and LLM that can handle confidential information.
Kobayashi: ChatGPT is a service provided on the cloud. Personal information managed by local governments is prohibited by law from being stored or processed in the cloud. In many cases, financial institutions are also not allowed to manage and process their data on the cloud because of its high confidentiality. In other words, if an organization or institution that handles highly confidential information wants to utilize LLM, it is necessary to build LLM dedicated to that organization to prevent information from leaking outside the organization. We are working on research and development of such LLM construction.
In addition, I believe that in order to utilize generative AI in a highly specialized company, it is necessary to have LLM with that expertise. In such cases, it is necessary to have LLM learn the knowledge of experts and the know-how of skilled technicians. I think these highly specialized LLM can be described as the “secret sauce” of know-how and knowledge gathered. I often hear people say that they want to utilize these “sauce” and at the same time protect it.
I am working on this project with the expectation that if we combine these various LLM with content such as images, audio, and video, we will be able to expand the range of AI applications even further than before.
—You mentioned earlier that LLM is one area of generative AI. As an expert in LLM research and development, what are your thoughts on the risks of LLM and generative AI today?
Kobayashi: Many of the risks associated with LLM and generative AI are directly related to trust and ethics. For example, issues of inaccurate or incorrect content output from AI, known as hallucination, can cause AI to be less trustworthy.
As for issues related to ethics, for example, fake images of famous politicians created by deepfakes could cause serious social problems. In another example that might occur in everyday life, LLM might give inappropriate answers, such as teaching a child how to make a bomb.
I believe that the evolution of AI is, in other words, getting closer to humans. Some people may expect that AI has become as capable as humans, which means that it has also acquired common sense and good intentions. However, this is an area where research is still lacking. So, from now on, AI-based systems will be required to ensure transparency in how the AI was constructed and accountability in showing how the AI derived its output. In the future, as AI becomes more capable, this requirement will increase.
Enjoy new AI society with expectations
—Fujitsu has long been developing explainable AI. Based on this perspective, what are your thoughts on the future of AI and its ideal use in society?
Kobayashi: We want to make sure that AI can be used safely as the foundation of society. With this in mind, Fujitsu is developing explainable AI. This is our mission as an AI developer and provider. If AI can clearly explain why it made such a decision and what kind of data it used to draw the conclusion, it can be used with confidence.
In addition to being explainable, transparency in terms of ethics will also be an important element of AI in the future. For example, whether the output of AI is guiding someone to some kind of ideology, or whether the information is selected arbitrarily. As explained earlier, as AI becomes more capable, transparency and accountability will become more important.
AI has many challenges, but on the other hand, there is no doubt that the future of AI has unlimited potential. About 20 years ago, the spread of search on the Internet led to a dramatic change in the sharing of knowledge with a variety of people. We can say that the same thing will happen with AI. It will be able to do things that could not be done before. I believe that society will change, with AI taking over the troublesome tasks that humans had to do.
In this sense, my message as a developer is not to be afraid of AI, but to see it with high expectations. Fujitsu’s mission is to develop AI for corporate customers, and to convey to them that AI has unlimited possibilities. We will also solve technical issues so that our customers can utilize AI safely and securely.
Generative AI, including ChatGPT, has had a huge impact on people around the world. Some people may worry or feel a certain amount of fear that Ai will be taking jobs away from humans. On the other hand, it is also true that AI has unlimited potential. Do not be afraid of AI. We hope that you will welcome the new AI society with the expectation of enjoying it.
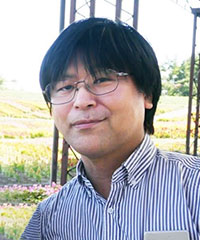
Kenichi Kobayashi
Research Director
Artificial Intelligence Laboratory
Fujitsu Research
Fujitsu Limited.
Related Information
- [Press Releases] Tokyo Tech, Tohoku University, Fujitsu, and RIKEN start collaboration to develop distributed training of Large Language Models : Fujitsu Global
- [Article] Redefining Innovation: How Generative AI is transforming the marketing landscape
- [Article] Solving Social Issues in the Space and Urban Transportation Fields by Simulating Electromagnetic Waves by supercomputer Fugaku